diff --git a/.compute b/.compute
index 63dea7a7..3e009cae 100644
--- a/.compute
+++ b/.compute
@@ -4,13 +4,13 @@ yes | apt-get install ffmpeg
yes | apt-get install espeak
yes | apt-get install tmux
yes | apt-get install zsh
-pip3 install https://download.pytorch.org/whl/cu100/torch-1.1.0-cp37-cp37m-linux_x86_64.whl
+# pip3 install https://download.pytorch.org/whl/cu100/torch-1.1.0-cp37-cp37m-linux_x86_64.whl
# wget https://www.dropbox.com/s/m8waow6b3ydpf6h/MozillaDataset.tar.gz?dl=0 -O /data/rw/home/mozilla.tar
wget https://www.dropbox.com/s/wqn5v3wkktw9lmo/install.sh?dl=0 -O install.sh
sudo sh install.sh
python3 setup.py develop
# cp -R ${USER_DIR}/GermanData ../tmp/
-python3 distribute.py --config_path config_libritts.json --data_path /data/rw/home/LibriTTS/train-clean-360/
+# python3 distribute.py --config_path config.json --data_path /data/ro/shared/data/keithito/LJSpeech-1.1/
# cp -R ${USER_DIR}/Mozilla_22050 ../tmp/
# python3 distribute.py --config_path config_tacotron_gst.json --data_path ../tmp/Mozilla_22050/
while true; do sleep 1000000; done
diff --git a/.travis/script b/.travis/script
index 4aa275be..41a17a4c 100755
--- a/.travis/script
+++ b/.travis/script
@@ -11,5 +11,7 @@ fi
if [[ "$TEST_SUITE" == "unittest" ]]; then
# Run tests on all pushes
+ pushd tts_namespace
python -m unittest
+ popd
fi
diff --git a/README.md b/README.md
index 068c1762..39e507e1 100644
--- a/README.md
+++ b/README.md
@@ -10,9 +10,9 @@ TTS includes two different model implementations which are based on [Tacotron](h
If you are new, you can also find [here](http://www.erogol.com/text-speech-deep-learning-architectures/) a brief post about TTS architectures and their comparisons.
## TTS Performance
-
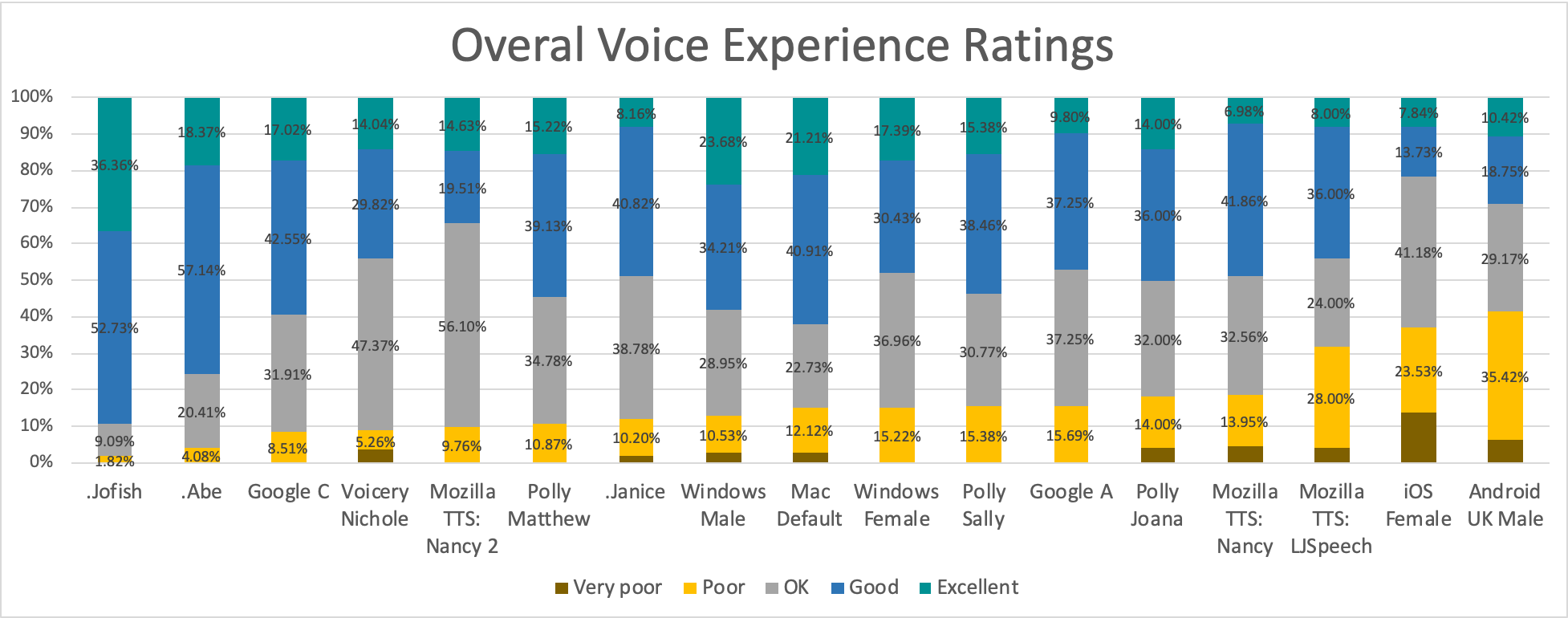
+
-[Details...](https://github.com/mozilla/TTS/issues/186)
+[Details...](https://github.com/mozilla/TTS/wiki/Mean-Opinion-Score-Results)
## Requirements and Installation
Highly recommended to use [miniconda](https://conda.io/miniconda.html) for easier installation.
diff --git a/__init__.py b/__init__.py
new file mode 100644
index 00000000..e69de29b
diff --git a/config.json b/config.json
index 807c4c60..4d56c3dc 100644
--- a/config.json
+++ b/config.json
@@ -1,6 +1,6 @@
{
- "run_name": "mozilla-no-loc-fattn-stopnet-sigmoid-loss_masking",
- "run_description": "using forward attention, with original prenet, loss masking,separate stopnet, sigmoid. Compare this with 4817. Pytorch DPP",
+ "run_name": "ljspeech",
+ "run_description": "gradual training with prenet frame size 1 + no maxout for cbhg + symmetric norm.",
"audio":{
// Audio processing parameters
@@ -16,8 +16,8 @@
"griffin_lim_iters": 60,// #griffin-lim iterations. 30-60 is a good range. Larger the value, slower the generation.
// Normalization parameters
"signal_norm": true, // normalize the spec values in range [0, 1]
- "symmetric_norm": false, // move normalization to range [-1, 1]
- "max_norm": 1, // scale normalization to range [-max_norm, max_norm] or [0, max_norm]
+ "symmetric_norm": true, // move normalization to range [-1, 1]
+ "max_norm": 4, // scale normalization to range [-max_norm, max_norm] or [0, max_norm]
"clip_norm": true, // clip normalized values into the range.
"mel_fmin": 0.0, // minimum freq level for mel-spec. ~50 for male and ~95 for female voices. Tune for dataset!!
"mel_fmax": 8000.0, // maximum freq level for mel-spec. Tune for dataset!!
@@ -31,44 +31,45 @@
"reinit_layers": [],
- "model": "Tacotron2", // one of the model in models/
+ "model": "Tacotron", // one of the model in models/
"grad_clip": 1, // upper limit for gradients for clipping.
"epochs": 1000, // total number of epochs to train.
"lr": 0.0001, // Initial learning rate. If Noam decay is active, maximum learning rate.
"lr_decay": false, // if true, Noam learning rate decaying is applied through training.
"warmup_steps": 4000, // Noam decay steps to increase the learning rate from 0 to "lr"
- "windowing": false, // Enables attention windowing. Used only in eval mode.
- "memory_size": 5, // ONLY TACOTRON - memory queue size used to queue network predictions to feed autoregressive connection. Useful if r < 5.
+ "memory_size": -1, // ONLY TACOTRON - size of the memory queue used fro storing last decoder predictions for auto-regression. If < 0, memory queue is disabled and decoder only uses the last prediction frame.
"attention_norm": "sigmoid", // softmax or sigmoid. Suggested to use softmax for Tacotron2 and sigmoid for Tacotron.
- "prenet_type": "original", // ONLY TACOTRON2 - "original" or "bn".
- "prenet_dropout": true, // ONLY TACOTRON2 - enable/disable dropout at prenet.
- "use_forward_attn": true, // ONLY TACOTRON2 - if it uses forward attention. In general, it aligns faster.
+ "prenet_type": "original", // "original" or "bn".
+ "prenet_dropout": true, // enable/disable dropout at prenet.
+ "windowing": false, // Enables attention windowing. Used only in eval mode.
+ "use_forward_attn": false, // if it uses forward attention. In general, it aligns faster.
"forward_attn_mask": false,
- "transition_agent": false, // ONLY TACOTRON2 - enable/disable transition agent of forward attention.
- "location_attn": false, // ONLY TACOTRON2 - enable_disable location sensitive attention. It is enabled for TACOTRON by default.
+ "transition_agent": false, // enable/disable transition agent of forward attention.
+ "location_attn": true, // enable_disable location sensitive attention. It is enabled for TACOTRON by default.
"loss_masking": true, // enable / disable loss masking against the sequence padding.
"enable_eos_bos_chars": false, // enable/disable beginning of sentence and end of sentence chars.
"stopnet": true, // Train stopnet predicting the end of synthesis.
"separate_stopnet": true, // Train stopnet seperately if 'stopnet==true'. It prevents stopnet loss to influence the rest of the model. It causes a better model, but it trains SLOWER.
"tb_model_param_stats": false, // true, plots param stats per layer on tensorboard. Might be memory consuming, but good for debugging.
- "batch_size": 32, // Batch size for training. Lower values than 32 might cause hard to learn attention.
+ "batch_size": 32, // Batch size for training. Lower values than 32 might cause hard to learn attention. It is overwritten by 'gradual_training'.
"eval_batch_size":16,
- "r": 1, // Number of frames to predict for step.
+ "r": 7, // Number of decoder frames to predict per iteration. Set the initial values if gradual training is enabled.
+ "gradual_training": [[0, 7, 32], [10000, 5, 32], [50000, 3, 32], [130000, 2, 16], [290000, 1, 8]], // ONLY TACOTRON - set gradual training steps [first_step, r, batch_size]. If it is null, gradual training is disabled.
"wd": 0.000001, // Weight decay weight.
"checkpoint": true, // If true, it saves checkpoints per "save_step"
- "save_step": 1000, // Number of training steps expected to save traning stats and checkpoints.
- "print_step": 10, // Number of steps to log traning on console.
+ "save_step": 10000, // Number of training steps expected to save traning stats and checkpoints.
+ "print_step": 25, // Number of steps to log traning on console.
"batch_group_size": 0, //Number of batches to shuffle after bucketing.
"run_eval": true,
"test_delay_epochs": 5, //Until attention is aligned, testing only wastes computation time.
"test_sentences_file": null, // set a file to load sentences to be used for testing. If it is null then we use default english sentences.
- "data_path": "/media/erogol/data_ssd/Data/Mozilla/", // DATASET-RELATED: can overwritten from command argument
- "meta_file_train": "metadata_train.txt", // DATASET-RELATED: metafile for training dataloader.
- "meta_file_val": "metadata_val.txt", // DATASET-RELATED: metafile for evaluation dataloader.
- "dataset": "mozilla", // DATASET-RELATED: one of TTS.dataset.preprocessors depending on your target dataset. Use "tts_cache" for pre-computed dataset by extract_features.py
- "min_seq_len": 0, // DATASET-RELATED: minimum text length to use in training
+ "data_path": "/home/erogol/Data/LJSpeech-1.1/", // DATASET-RELATED: can overwritten from command argument
+ "meta_file_train": "metadata_train.csv", // DATASET-RELATED: metafile for training dataloader.
+ "meta_file_val": "metadata_val.csv", // DATASET-RELATED: metafile for evaluation dataloader.
+ "dataset": "ljspeech", // DATASET-RELATED: one of TTS.dataset.preprocessors depending on your target dataset. Use "tts_cache" for pre-computed dataset by extract_features.py
+ "min_seq_len": 6, // DATASET-RELATED: minimum text length to use in training
"max_seq_len": 150, // DATASET-RELATED: maximum text length
"output_path": "../keep/", // DATASET-RELATED: output path for all training outputs.
"num_loader_workers": 4, // number of training data loader processes. Don't set it too big. 4-8 are good values.
@@ -77,6 +78,7 @@
"use_phonemes": true, // use phonemes instead of raw characters. It is suggested for better pronounciation.
"phoneme_language": "en-us", // depending on your target language, pick one from https://github.com/bootphon/phonemizer#languages
"text_cleaner": "phoneme_cleaners",
- "use_speaker_embedding": false // whether to use additional embeddings for separate speakers
+ "use_speaker_embedding": false, // use speaker embedding to enable multi-speaker learning.
+ "style_wav_for_test": null // path to style wav file to be used in TacotronGST inference.
}
diff --git a/config_kusal.json b/config_kusal.json
deleted file mode 100644
index 696171f0..00000000
--- a/config_kusal.json
+++ /dev/null
@@ -1,41 +0,0 @@
-{
- "model_name": "TTS-larger-kusal",
- "audio_processor": "audio",
- "num_mels": 80,
- "num_freq": 1025,
- "sample_rate": 22000,
- "frame_length_ms": 50,
- "frame_shift_ms": 12.5,
- "preemphasis": 0.97,
- "min_mel_freq": 125,
- "max_mel_freq": 7600,
- "min_level_db": -100,
- "ref_level_db": 20,
- "embedding_size": 256,
- "text_cleaner": "english_cleaners",
-
- "epochs": 1000,
- "lr": 0.002,
- "lr_decay": 0.5,
- "decay_step": 100000,
- "warmup_steps": 4000,
- "batch_size": 32,
- "eval_batch_size":-1,
- "r": 5,
-
- "griffin_lim_iters": 60,
- "power": 1.5,
-
- "num_loader_workers": 8,
-
- "checkpoint": true,
- "save_step": 25000,
- "print_step": 10,
- "run_eval": false,
- "data_path": "/snakepit/shared/data/mycroft/kusal/",
- "meta_file_train": "prompts.txt",
- "meta_file_val": null,
- "dataset": "Kusal",
- "min_seq_len": 0,
- "output_path": "../keep/"
-}
\ No newline at end of file
diff --git a/config_libritts.json b/config_libritts.json
deleted file mode 100644
index f9a752ec..00000000
--- a/config_libritts.json
+++ /dev/null
@@ -1,82 +0,0 @@
-{
- "run_name": "libritts-360",
- "run_description": "LibriTTS 360 clean with multi speaker embedding.",
-
- "audio":{
- // Audio processing parameters
- "num_mels": 80, // size of the mel spec frame.
- "num_freq": 1025, // number of stft frequency levels. Size of the linear spectogram frame.
- "sample_rate": 16000, // DATASET-RELATED: wav sample-rate. If different than the original data, it is resampled.
- "frame_length_ms": 50, // stft window length in ms.
- "frame_shift_ms": 12.5, // stft window hop-lengh in ms.
- "preemphasis": 0.98, // pre-emphasis to reduce spec noise and make it more structured. If 0.0, no -pre-emphasis.
- "min_level_db": -100, // normalization range
- "ref_level_db": 20, // reference level db, theoretically 20db is the sound of air.
- "power": 1.5, // value to sharpen wav signals after GL algorithm.
- "griffin_lim_iters": 60,// #griffin-lim iterations. 30-60 is a good range. Larger the value, slower the generation.
- // Normalization parameters
- "signal_norm": true, // normalize the spec values in range [0, 1]
- "symmetric_norm": false, // move normalization to range [-1, 1]
- "max_norm": 1, // scale normalization to range [-max_norm, max_norm] or [0, max_norm]
- "clip_norm": true, // clip normalized values into the range.
- "mel_fmin": 0.0, // minimum freq level for mel-spec. ~50 for male and ~95 for female voices. Tune for dataset!!
- "mel_fmax": 8000.0, // maximum freq level for mel-spec. Tune for dataset!!
- "do_trim_silence": true // enable trimming of slience of audio as you load it. LJspeech (false), TWEB (false), Nancy (true)
- },
-
- "distributed":{
- "backend": "nccl",
- "url": "tcp:\/\/localhost:54321"
- },
-
- "reinit_layers": [],
-
- "model": "Tacotron2", // one of the model in models/
- "grad_clip": 1, // upper limit for gradients for clipping.
- "epochs": 1000, // total number of epochs to train.
- "lr": 0.0001, // Initial learning rate. If Noam decay is active, maximum learning rate.
- "lr_decay": false, // if true, Noam learning rate decaying is applied through training.
- "warmup_steps": 4000, // Noam decay steps to increase the learning rate from 0 to "lr"
- "memory_size": 5, // ONLY TACOTRON - memory queue size used to queue network predictions to feed autoregressive connection. Useful if r < 5.
- "attention_norm": "sigmoid", // softmax or sigmoid. Suggested to use softmax for Tacotron2 and sigmoid for Tacotron.
- "prenet_type": "original", // ONLY TACOTRON2 - "original" or "bn".
- "prenet_dropout": true, // ONLY TACOTRON2 - enable/disable dropout at prenet.
- "windowing": false, // Enables attention windowing. Used only in eval mode.
- "use_forward_attn": false, // ONLY TACOTRON2 - if it uses forward attention. In general, it aligns faster.
- "forward_attn_mask": false,
- "transition_agent": false, // ONLY TACOTRON2 - enable/disable transition agent of forward attention.
- "location_attn": true, // ONLY TACOTRON2 - enable_disable location sensitive attention. It is enabled for TACOTRON by default.
- "loss_masking": true, // enable / disable loss masking against the sequence padding.
- "enable_eos_bos_chars": false, // enable/disable beginning of sentence and end of sentence chars.
- "stopnet": true, // Train stopnet predicting the end of synthesis.
- "separate_stopnet": true, // Train stopnet seperately if 'stopnet==true'. It prevents stopnet loss to influence the rest of the model. It causes a better model, but it trains SLOWER.
- "tb_model_param_stats": true, // true, plots param stats per layer on tensorboard. Might be memory consuming, but good for debugging.
-
- "batch_size": 24, // Batch size for training. Lower values than 32 might cause hard to learn attention.
- "eval_batch_size":16,
- "r": 1, // Number of frames to predict for step.
- "wd": 0.000001, // Weight decay weight.
- "checkpoint": true, // If true, it saves checkpoints per "save_step"
- "save_step": 1000, // Number of training steps expected to save traning stats and checkpoints.
- "print_step": 10, // Number of steps to log traning on console.
- "batch_group_size": 0, //Number of batches to shuffle after bucketing.
-
- "run_eval": true,
- "test_delay_epochs": 5, //Until attention is aligned, testing only wastes computation time.
- "test_sentences_file": null, // set a file to load sentences to be used for testing. If it is null then we use default english sentences.
- "data_path": "/home/erogol/Data/Libri-TTS/train-clean-360/", // DATASET-RELATED: can overwritten from command argument
- "meta_file_train": null, // DATASET-RELATED: metafile for training dataloader.
- "meta_file_val": null, // DATASET-RELATED: metafile for evaluation dataloader.
- "dataset": "libri_tts", // DATASET-RELATED: one of TTS.dataset.preprocessors depending on your target dataset. Use "tts_cache" for pre-computed dataset by extract_features.py
- "min_seq_len": 6, // DATASET-RELATED: minimum text length to use in training
- "max_seq_len": 150, // DATASET-RELATED: maximum text length
- "output_path": "/media/erogol/data_ssd/Models/libri_tts/", // DATASET-RELATED: output path for all training outputs.
- "num_loader_workers": 12, // number of training data loader processes. Don't set it too big. 4-8 are good values.
- "num_val_loader_workers": 4, // number of evaluation data loader processes.
- "phoneme_cache_path": "mozilla_us_phonemes", // phoneme computation is slow, therefore, it caches results in the given folder.
- "use_phonemes": true, // use phonemes instead of raw characters. It is suggested for better pronounciation.
- "phoneme_language": "en-us", // depending on your target language, pick one from https://github.com/bootphon/phonemizer#languages
- "text_cleaner": "phoneme_cleaners",
- "use_speaker_embedding": true
-}
-
diff --git a/config_tacotron.json b/config_tacotron.json
index 127a4b3d..92ee3909 100644
--- a/config_tacotron.json
+++ b/config_tacotron.json
@@ -42,10 +42,10 @@
"attention_norm": "sigmoid", // softmax or sigmoid. Suggested to use softmax for Tacotron2 and sigmoid for Tacotron.
"prenet_type": "original", // "original" or "bn".
"prenet_dropout": true, // enable/disable dropout at prenet.
- "use_forward_attn": true, // if it uses forward attention. In general, it aligns faster.
+ "use_forward_attn": true, // enable/disable forward attention. In general, it aligns faster.
"forward_attn_mask": false, // Apply forward attention mask af inference to prevent bad modes. Try it if your model does not align well.
"transition_agent": true, // enable/disable transition agent of forward attention.
- "location_attn": false, // enable_disable location sensitive attention. It is enabled for TACOTRON by default.
+ "location_attn": false, // enable_disable location sensitive attention.
"loss_masking": true, // enable / disable loss masking against the sequence padding.
"enable_eos_bos_chars": false, // enable/disable beginning of sentence and end of sentence chars.
"stopnet": true, // Train stopnet predicting the end of synthesis.
diff --git a/config_tacotron2.json b/config_tacotron2.json
index fd188d20..02b4341b 100644
--- a/config_tacotron2.json
+++ b/config_tacotron2.json
@@ -39,12 +39,12 @@
"warmup_steps": 4000, // Noam decay steps to increase the learning rate from 0 to "lr"
"memory_size": 5, // ONLY TACOTRON - memory queue size used to queue network predictions to feed autoregressive connection. Useful if r < 5.
"attention_norm": "sigmoid", // softmax or sigmoid. Suggested to use softmax for Tacotron2 and sigmoid for Tacotron.
- "prenet_type": "original", // ONLY TACOTRON2 - "original" or "bn".
- "prenet_dropout": true, // ONLY TACOTRON2 - enable/disable dropout at prenet.
- "use_forward_attn": true, // ONLY TACOTRON2 - if it uses forward attention. In general, it aligns faster.
+ "prenet_type": "original", // "original" or "bn".
+ "prenet_dropout": true, // enable/disable dropout at prenet.
+ "use_forward_attn": true, // enable/disable forward attention. In general, it aligns faster.
"forward_attn_mask": false, // Apply forward attention mask af inference to prevent bad modes. Try it if your model does not align well.
- "transition_agent": false, // ONLY TACOTRON2 - enable/disable transition agent of forward attention.
- "location_attn": false, // ONLY TACOTRON2 - enable_disable location sensitive attention. It is enabled for TACOTRON by default.
+ "transition_agent": false, // enable/disable transition agent of forward attention.
+ "location_attn": false, // enable_disable location sensitive attention. It is enabled for TACOTRON by default.
"loss_masking": true, // enable / disable loss masking against the sequence padding.
"enable_eos_bos_chars": false, // enable/disable beginning of sentence and end of sentence chars.
"stopnet": true, // Train stopnet predicting the end of synthesis.
diff --git a/config_tacotron_de.json b/config_tacotron_de.json
index 834bfed4..fc3efbec 100644
--- a/config_tacotron_de.json
+++ b/config_tacotron_de.json
@@ -40,12 +40,12 @@
"windowing": false, // Enables attention windowing. Used only in eval mode.
"memory_size": 5, // ONLY TACOTRON - memory queue size used to queue network predictions to feed autoregressive connection. Useful if r < 5.
"attention_norm": "sigmoid", // softmax or sigmoid. Suggested to use softmax for Tacotron2 and sigmoid for Tacotron.
- "prenet_type": "original", // ONLY TACOTRON2 - "original" or "bn".
- "prenet_dropout": true, // ONLY TACOTRON2 - enable/disable dropout at prenet.
- "use_forward_attn": false, // ONLY TACOTRON2 - if it uses forward attention. In general, it aligns faster.
- "transition_agent": false, // ONLY TACOTRON2 - enable/disable transition agent of forward attention.
- "forward_attn_mask": false,
- "location_attn": true, // ONLY TACOTRON2 - enable_disable location sensitive attention. It is enabled for TACOTRON by default.
+ "prenet_type": "original", // "original" or "bn".
+ "prenet_dropout": true, // enable/disable dropout at prenet.
+ "use_forward_attn": false, // enable/disable forward attention. In general, it aligns faster.
+ "transition_agent": false, // enable/disable transition agent of forward attention.
+ "forward_attn_mask": false, // Apply forward attention mask at inference to prevent bad modes. Try it if your model does not align well.
+ "location_attn": true, // enable_disable location sensitive attention. It is enabled for TACOTRON by default.
"loss_masking": true, // enable / disable loss masking against the sequence padding.
"enable_eos_bos_chars": false, // enable/disable beginning of sentence and end of sentence chars.
"stopnet": true, // Train stopnet predicting the end of synthesis.
diff --git a/config_tacotron_gst.json b/config_tacotron_gst.json
index 98fafa54..e56c85dd 100644
--- a/config_tacotron_gst.json
+++ b/config_tacotron_gst.json
@@ -42,8 +42,8 @@
"attention_norm": "sigmoid", // softmax or sigmoid. Suggested to use softmax for Tacotron2 and sigmoid for Tacotron.
"prenet_type": "original", // "original" or "bn".
"prenet_dropout": true, // enable/disable dropout at prenet.
- "use_forward_attn": true, // if it uses forward attention. In general, it aligns faster.
- "forward_attn_mask": false, // Apply forward attention mask af inference to prevent bad modes. Try it if your model does not align well.
+ "use_forward_attn": true, // enable/disable forward attention. In general, it aligns faster.
+ "forward_attn_mask": false, // Apply forward attention mask at inference to prevent bad modes. Try it if your model does not align well.
"transition_agent": false, // enable/disable transition agent of forward attention.
"location_attn": false, // enable_disable location sensitive attention. It is enabled for TACOTRON by default.
"loss_masking": true, // enable / disable loss masking against the sequence padding.
@@ -77,6 +77,7 @@
"use_phonemes": true, // use phonemes instead of raw characters. It is suggested for better pronounciation.
"phoneme_language": "en-us", // depending on your target language, pick one from https://github.com/bootphon/phonemizer#languages
"text_cleaner": "phoneme_cleaners",
- "use_speaker_embedding": false // whether to use additional embeddings for separate speakers
+ "use_speaker_embedding": false, // whether to use additional embeddings for separate speakers
+ "style_wav_for_test": null // path to wav for styling the inference tests when using GST
}
\ No newline at end of file
diff --git a/datasets/TTSDataset.py b/datasets/TTSDataset.py
index ecf8e9ea..cbb4bf97 100644
--- a/datasets/TTSDataset.py
+++ b/datasets/TTSDataset.py
@@ -5,8 +5,8 @@ import torch
import random
from torch.utils.data import Dataset
-from utils.text import text_to_sequence, phoneme_to_sequence, pad_with_eos_bos
-from utils.data import prepare_data, prepare_tensor, prepare_stop_target
+from TTS.utils.text import text_to_sequence, phoneme_to_sequence, pad_with_eos_bos
+from TTS.utils.data import prepare_data, prepare_tensor, prepare_stop_target
class MyDataset(Dataset):
@@ -102,7 +102,7 @@ class MyDataset(Dataset):
cache_path)
if self.enable_eos_bos:
phonemes = pad_with_eos_bos(phonemes)
-
+ phonemes = np.asarray(phonemes, dtype=np.int32)
return phonemes
def load_data(self, idx):
diff --git a/datasets/preprocess.py b/datasets/preprocess.py
index 9dd7a610..e5f4e1a2 100644
--- a/datasets/preprocess.py
+++ b/datasets/preprocess.py
@@ -75,21 +75,19 @@ def mailabs(root_path, meta_files=None):
speaker_regex = re.compile("by_book/(male|female)/(?P[^/]+)/")
if meta_files is None:
csv_files = glob(root_path+"/**/metadata.csv", recursive=True)
- folders = [os.path.dirname(f) for f in csv_files]
else:
csv_files = meta_files
- folders = [f.strip().split("by_book")[1][1:] for f in csv_files]
# meta_files = [f.strip() for f in meta_files.split(",")]
items = []
- for idx, csv_file in enumerate(csv_files):
+ for csv_file in csv_files:
+ txt_file = os.path.join(root_path, csv_file)
+ folder = os.path.dirname(txt_file)
# determine speaker based on folder structure...
- speaker_name_match = speaker_regex.search(csv_file)
+ speaker_name_match = speaker_regex.search(txt_file)
if speaker_name_match is None:
continue
speaker_name = speaker_name_match.group("speaker_name")
print(" | > {}".format(csv_file))
- folder = folders[idx]
- txt_file = os.path.join(root_path, csv_file)
with open(txt_file, 'r') as ttf:
for line in ttf:
cols = line.split('|')
diff --git a/distribute.py b/distribute.py
index 22c27b1c..f65fbe71 100644
--- a/distribute.py
+++ b/distribute.py
@@ -9,7 +9,7 @@ import torch.distributed as dist
from torch.utils.data.sampler import Sampler
from torch.autograd import Variable
from torch._utils import _flatten_dense_tensors, _unflatten_dense_tensors
-from utils.generic_utils import load_config, create_experiment_folder
+from TTS.utils.generic_utils import load_config, create_experiment_folder
class DistributedSampler(Sampler):
diff --git a/layers/common_layers.py b/layers/common_layers.py
index 2edf0dab..d5836a9f 100644
--- a/layers/common_layers.py
+++ b/layers/common_layers.py
@@ -108,19 +108,19 @@ class LocationLayer(nn.Module):
class Attention(nn.Module):
# Pylint gets confused by PyTorch conventions here
#pylint: disable=attribute-defined-outside-init
- def __init__(self, attention_rnn_dim, embedding_dim, attention_dim,
+ def __init__(self, query_dim, embedding_dim, attention_dim,
location_attention, attention_location_n_filters,
attention_location_kernel_size, windowing, norm, forward_attn,
trans_agent, forward_attn_mask):
super(Attention, self).__init__()
self.query_layer = Linear(
- attention_rnn_dim, attention_dim, bias=False, init_gain='tanh')
+ query_dim, attention_dim, bias=False, init_gain='tanh')
self.inputs_layer = Linear(
embedding_dim, attention_dim, bias=False, init_gain='tanh')
self.v = Linear(attention_dim, 1, bias=True)
if trans_agent:
self.ta = nn.Linear(
- attention_rnn_dim + embedding_dim, 1, bias=True)
+ query_dim + embedding_dim, 1, bias=True)
if location_attention:
self.location_layer = LocationLayer(
attention_dim,
@@ -201,16 +201,17 @@ class Attention(nn.Module):
self.win_idx = torch.argmax(attention, 1).long()[0].item()
return attention
- def apply_forward_attention(self, inputs, alignment, query):
+ def apply_forward_attention(self, alignment):
# forward attention
- prev_alpha = F.pad(self.alpha[:, :-1].clone(),
- (1, 0, 0, 0)).to(inputs.device)
+ fwd_shifted_alpha = F.pad(self.alpha[:, :-1].clone().to(alignment.device),
+ (1, 0, 0, 0))
# compute transition potentials
- alpha = (((1 - self.u) * self.alpha.clone().to(inputs.device) +
- self.u * prev_alpha) + 1e-8) * alignment
+ alpha = ((1 - self.u) * self.alpha
+ + self.u * fwd_shifted_alpha
+ + 1e-8) * alignment
# force incremental alignment
if not self.training and self.forward_attn_mask:
- _, n = prev_alpha.max(1)
+ _, n = fwd_shifted_alpha.max(1)
val, n2 = alpha.max(1)
for b in range(alignment.shape[0]):
alpha[b, n[b] + 3:] = 0
@@ -220,30 +221,24 @@ class Attention(nn.Module):
alpha[b,
(n[b] - 2
)] = 0.01 * val[b] # smoothing factor for the prev step
- # compute attention weights
- self.alpha = alpha / alpha.sum(dim=1).unsqueeze(1)
- # compute context
- context = torch.bmm(self.alpha.unsqueeze(1), inputs)
- context = context.squeeze(1)
- # compute transition agent
- if self.trans_agent:
- ta_input = torch.cat([context, query.squeeze(1)], dim=-1)
- self.u = torch.sigmoid(self.ta(ta_input))
- return context, self.alpha
+ # renormalize attention weights
+ alpha = alpha / alpha.sum(dim=1, keepdim=True)
+ return alpha
- def forward(self, attention_hidden_state, inputs, processed_inputs, mask):
+ def forward(self, query, inputs, processed_inputs, mask):
if self.location_attention:
- attention, processed_query = self.get_location_attention(
- attention_hidden_state, processed_inputs)
+ attention, _ = self.get_location_attention(
+ query, processed_inputs)
else:
- attention, processed_query = self.get_attention(
- attention_hidden_state, processed_inputs)
+ attention, _ = self.get_attention(
+ query, processed_inputs)
# apply masking
if mask is not None:
- attention.data.masked_fill_(1 - mask, self._mask_value)
+ attention.data.masked_fill_(~mask, self._mask_value)
# apply windowing - only in eval mode
if not self.training and self.windowing:
attention = self.apply_windowing(attention, inputs)
+
# normalize attention values
if self.norm == "softmax":
alignment = torch.softmax(attention, dim=-1)
@@ -252,15 +247,22 @@ class Attention(nn.Module):
attention).sum(
dim=1, keepdim=True)
else:
- raise RuntimeError("Unknown value for attention norm type")
+ raise ValueError("Unknown value for attention norm type")
+
if self.location_attention:
self.update_location_attention(alignment)
+
# apply forward attention if enabled
if self.forward_attn:
- context, self.attention_weights = self.apply_forward_attention(
- inputs, alignment, attention_hidden_state)
- else:
- context = torch.bmm(alignment.unsqueeze(1), inputs)
- context = context.squeeze(1)
- self.attention_weights = alignment
+ alignment = self.apply_forward_attention(alignment)
+ self.alpha = alignment
+
+ context = torch.bmm(alignment.unsqueeze(1), inputs)
+ context = context.squeeze(1)
+ self.attention_weights = alignment
+
+ # compute transition agent
+ if self.forward_attn and self.trans_agent:
+ ta_input = torch.cat([context, query.squeeze(1)], dim=-1)
+ self.u = torch.sigmoid(self.ta(ta_input))
return context
diff --git a/layers/losses.py b/layers/losses.py
index 5a95c0fe..a6bf95d3 100644
--- a/layers/losses.py
+++ b/layers/losses.py
@@ -1,6 +1,6 @@
from torch import nn
from torch.nn import functional
-from utils.generic_utils import sequence_mask
+from TTS.utils.generic_utils import sequence_mask
class L1LossMasked(nn.Module):
diff --git a/layers/tacotron.py b/layers/tacotron.py
index b71ddbc3..788e5230 100644
--- a/layers/tacotron.py
+++ b/layers/tacotron.py
@@ -135,9 +135,6 @@ class CBHG(nn.Module):
])
# max pooling of conv bank, with padding
# TODO: try average pooling OR larger kernel size
- self.max_pool1d = nn.Sequential(
- nn.ConstantPad1d([0, 1], value=0),
- nn.MaxPool1d(kernel_size=2, stride=1, padding=0))
out_features = [K * conv_bank_features] + conv_projections[:-1]
activations = [self.relu] * (len(conv_projections) - 1)
activations += [None]
@@ -186,7 +183,6 @@ class CBHG(nn.Module):
outs.append(out)
x = torch.cat(outs, dim=1)
assert x.size(1) == self.conv_bank_features * len(self.conv1d_banks)
- x = self.max_pool1d(x)
for conv1d in self.conv1d_projections:
x = conv1d(x)
# (B, T_in, hid_feature)
@@ -270,59 +266,57 @@ class Decoder(nn.Module):
memory_size (int): size of the past window. if <= 0 memory_size = r
TODO: arguments
"""
+
# Pylint gets confused by PyTorch conventions here
#pylint: disable=attribute-defined-outside-init
def __init__(self, in_features, memory_dim, r, memory_size, attn_windowing,
attn_norm, prenet_type, prenet_dropout, forward_attn,
- trans_agent, forward_attn_mask, location_attn, separate_stopnet):
+ trans_agent, forward_attn_mask, location_attn,
+ separate_stopnet):
super(Decoder, self).__init__()
+ self.r_init = r
self.r = r
self.in_features = in_features
self.max_decoder_steps = 500
+ self.use_memory_queue = memory_size > 0
self.memory_size = memory_size if memory_size > 0 else r
self.memory_dim = memory_dim
self.separate_stopnet = separate_stopnet
+ self.query_dim = 256
# memory -> |Prenet| -> processed_memory
self.prenet = Prenet(
- memory_dim * self.memory_size,
+ memory_dim * self.memory_size if self.use_memory_queue else memory_dim,
prenet_type,
prenet_dropout,
out_features=[256, 128])
# processed_inputs, processed_memory -> |Attention| -> Attention, attention, RNN_State
- self.attention_rnn = nn.GRUCell(in_features + 128, 256)
- self.attention_layer = Attention(attention_rnn_dim=256,
- embedding_dim=in_features,
- attention_dim=128,
- location_attention=location_attn,
- attention_location_n_filters=32,
- attention_location_kernel_size=31,
- windowing=attn_windowing,
- norm=attn_norm,
- forward_attn=forward_attn,
- trans_agent=trans_agent,
- forward_attn_mask=forward_attn_mask)
+ # attention_rnn generates queries for the attention mechanism
+ self.attention_rnn = nn.GRUCell(in_features + 128, self.query_dim)
+
+ self.attention = Attention(query_dim=self.query_dim,
+ embedding_dim=in_features,
+ attention_dim=128,
+ location_attention=location_attn,
+ attention_location_n_filters=32,
+ attention_location_kernel_size=31,
+ windowing=attn_windowing,
+ norm=attn_norm,
+ forward_attn=forward_attn,
+ trans_agent=trans_agent,
+ forward_attn_mask=forward_attn_mask)
# (processed_memory | attention context) -> |Linear| -> decoder_RNN_input
self.project_to_decoder_in = nn.Linear(256 + in_features, 256)
# decoder_RNN_input -> |RNN| -> RNN_state
self.decoder_rnns = nn.ModuleList(
[nn.GRUCell(256, 256) for _ in range(2)])
# RNN_state -> |Linear| -> mel_spec
- self.proj_to_mel = nn.Linear(256, memory_dim * r)
+ self.proj_to_mel = nn.Linear(256, memory_dim * self.r_init)
# learn init values instead of zero init.
- self.attention_rnn_init = nn.Embedding(1, 256)
- self.memory_init = nn.Embedding(1, self.memory_size * memory_dim)
- self.decoder_rnn_inits = nn.Embedding(2, 256)
- self.stopnet = StopNet(256 + memory_dim * r)
- # self.init_layers()
+ self.stopnet = StopNet(256 + memory_dim * self.r_init)
- def init_layers(self):
- torch.nn.init.xavier_uniform_(
- self.project_to_decoder_in.weight,
- gain=torch.nn.init.calculate_gain('linear'))
- torch.nn.init.xavier_uniform_(
- self.proj_to_mel.weight,
- gain=torch.nn.init.calculate_gain('linear'))
+ def set_r(self, new_r):
+ self.r = new_r
def _reshape_memory(self, memory):
"""
@@ -344,21 +338,19 @@ class Decoder(nn.Module):
B = inputs.size(0)
T = inputs.size(1)
# go frame as zeros matrix
- self.memory_input = self.memory_init(inputs.data.new_zeros(B).long())
-
+ if self.use_memory_queue:
+ self.memory_input = torch.zeros(B, self.memory_dim * self.memory_size, device=inputs.device)
+ else:
+ self.memory_input = torch.zeros(B, self.memory_dim, device=inputs.device)
# decoder states
- self.attention_rnn_hidden = self.attention_rnn_init(
- inputs.data.new_zeros(B).long())
+ self.attention_rnn_hidden = torch.zeros(B, 256, device=inputs.device)
self.decoder_rnn_hiddens = [
- self.decoder_rnn_inits(inputs.data.new_tensor([idx] * B).long())
+ torch.zeros(B, 256, device=inputs.device)
for idx in range(len(self.decoder_rnns))
]
- self.current_context_vec = inputs.data.new(B, self.in_features).zero_()
- # attention states
- self.attention = inputs.data.new(B, T).zero_()
- self.attention_cum = inputs.data.new(B, T).zero_()
+ self.context_vec = inputs.data.new(B, self.in_features).zero_()
# cache attention inputs
- self.processed_inputs = self.attention_layer.inputs_layer(inputs)
+ self.processed_inputs = self.attention.inputs_layer(inputs)
def _parse_outputs(self, outputs, attentions, stop_tokens):
# Back to batch first
@@ -371,12 +363,15 @@ class Decoder(nn.Module):
# Prenet
processed_memory = self.prenet(self.memory_input)
# Attention RNN
- self.attention_rnn_hidden = self.attention_rnn(torch.cat((processed_memory, self.current_context_vec), -1), self.attention_rnn_hidden)
- self.current_context_vec = self.attention_layer(self.attention_rnn_hidden, inputs, self.processed_inputs, mask)
+ self.attention_rnn_hidden = self.attention_rnn(
+ torch.cat((processed_memory, self.context_vec), -1),
+ self.attention_rnn_hidden)
+ self.context_vec = self.attention(
+ self.attention_rnn_hidden, inputs, self.processed_inputs, mask)
# Concat RNN output and attention context vector
decoder_input = self.project_to_decoder_in(
- torch.cat((self.attention_rnn_hidden, self.current_context_vec),
- -1))
+ torch.cat((self.attention_rnn_hidden, self.context_vec), -1))
+
# Pass through the decoder RNNs
for idx in range(len(self.decoder_rnns)):
self.decoder_rnn_hiddens[idx] = self.decoder_rnns[idx](
@@ -384,28 +379,33 @@ class Decoder(nn.Module):
# Residual connection
decoder_input = self.decoder_rnn_hiddens[idx] + decoder_input
decoder_output = decoder_input
- del decoder_input
+
# predict mel vectors from decoder vectors
output = self.proj_to_mel(decoder_output)
- output = torch.sigmoid(output)
+ # output = torch.sigmoid(output)
# predict stop token
stopnet_input = torch.cat([decoder_output, output], -1)
- del decoder_output
if self.separate_stopnet:
stop_token = self.stopnet(stopnet_input.detach())
else:
stop_token = self.stopnet(stopnet_input)
- return output, stop_token, self.attention_layer.attention_weights
+ output = output[:, : self.r * self.memory_dim]
+ return output, stop_token, self.attention.attention_weights
- def _update_memory_queue(self, new_memory):
- if self.memory_size > 0 and new_memory.shape[-1] < self.memory_size:
- self.memory_input = torch.cat([
- self.memory_input[:, self.r * self.memory_dim:].clone(),
- new_memory
- ],
- dim=-1)
+ def _update_memory_input(self, new_memory):
+ if self.use_memory_queue:
+ if self.memory_size > self.r:
+ # memory queue size is larger than number of frames per decoder iter
+ self.memory_input = torch.cat([
+ new_memory, self.memory_input[:, :(
+ self.memory_size - self.r) * self.memory_dim].clone()
+ ], dim=-1)
+ else:
+ # memory queue size smaller than number of frames per decoder iter
+ self.memory_input = new_memory[:, :self.memory_size * self.memory_dim]
else:
- self.memory_input = new_memory
+ # use only the last frame prediction
+ self.memory_input = new_memory[:, :self.memory_dim]
def forward(self, inputs, memory, mask):
"""
@@ -427,11 +427,11 @@ class Decoder(nn.Module):
stop_tokens = []
t = 0
self._init_states(inputs)
- self.attention_layer.init_states(inputs)
+ self.attention.init_states(inputs)
while len(outputs) < memory.size(0):
if t > 0:
new_memory = memory[t - 1]
- self._update_memory_queue(new_memory)
+ self._update_memory_input(new_memory)
output, stop_token, attention = self.decode(inputs, mask)
outputs += [output]
attentions += [attention]
@@ -453,12 +453,12 @@ class Decoder(nn.Module):
stop_tokens = []
t = 0
self._init_states(inputs)
- self.attention_layer.init_win_idx()
- self.attention_layer.init_states(inputs)
+ self.attention.init_win_idx()
+ self.attention.init_states(inputs)
while True:
if t > 0:
new_memory = outputs[-1]
- self._update_memory_queue(new_memory)
+ self._update_memory_input(new_memory)
output, stop_token, attention = self.decode(inputs, None)
stop_token = torch.sigmoid(stop_token.data)
outputs += [output]
diff --git a/layers/tacotron2.py b/layers/tacotron2.py
index 802f158e..358d1807 100644
--- a/layers/tacotron2.py
+++ b/layers/tacotron2.py
@@ -104,7 +104,7 @@ class Decoder(nn.Module):
self.r = r
self.encoder_embedding_dim = in_features
self.separate_stopnet = separate_stopnet
- self.attention_rnn_dim = 1024
+ self.query_dim = 1024
self.decoder_rnn_dim = 1024
self.prenet_dim = 256
self.max_decoder_steps = 1000
@@ -117,21 +117,21 @@ class Decoder(nn.Module):
[self.prenet_dim, self.prenet_dim], bias=False)
self.attention_rnn = nn.LSTMCell(self.prenet_dim + in_features,
- self.attention_rnn_dim)
+ self.query_dim)
- self.attention_layer = Attention(attention_rnn_dim=self.attention_rnn_dim,
- embedding_dim=in_features,
- attention_dim=128,
- location_attention=location_attn,
- attention_location_n_filters=32,
- attention_location_kernel_size=31,
- windowing=attn_win,
- norm=attn_norm,
- forward_attn=forward_attn,
- trans_agent=trans_agent,
- forward_attn_mask=forward_attn_mask)
+ self.attention = Attention(query_dim=self.query_dim,
+ embedding_dim=in_features,
+ attention_dim=128,
+ location_attention=location_attn,
+ attention_location_n_filters=32,
+ attention_location_kernel_size=31,
+ windowing=attn_win,
+ norm=attn_norm,
+ forward_attn=forward_attn,
+ trans_agent=trans_agent,
+ forward_attn_mask=forward_attn_mask)
- self.decoder_rnn = nn.LSTMCell(self.attention_rnn_dim + in_features,
+ self.decoder_rnn = nn.LSTMCell(self.query_dim + in_features,
self.decoder_rnn_dim, 1)
self.linear_projection = Linear(self.decoder_rnn_dim + in_features,
@@ -145,7 +145,7 @@ class Decoder(nn.Module):
bias=True,
init_gain='sigmoid'))
- self.attention_rnn_init = nn.Embedding(1, self.attention_rnn_dim)
+ self.attention_rnn_init = nn.Embedding(1, self.query_dim)
self.go_frame_init = nn.Embedding(1, self.mel_channels * r)
self.decoder_rnn_inits = nn.Embedding(1, self.decoder_rnn_dim)
self.memory_truncated = None
@@ -160,10 +160,10 @@ class Decoder(nn.Module):
# T = inputs.size(1)
if not keep_states:
- self.attention_hidden = self.attention_rnn_init(
+ self.query = self.attention_rnn_init(
inputs.data.new_zeros(B).long())
- self.attention_cell = Variable(
- inputs.data.new(B, self.attention_rnn_dim).zero_())
+ self.attention_rnn_cell_state = Variable(
+ inputs.data.new(B, self.query_dim).zero_())
self.decoder_hidden = self.decoder_rnn_inits(
inputs.data.new_zeros(B).long())
@@ -174,7 +174,7 @@ class Decoder(nn.Module):
inputs.data.new(B, self.encoder_embedding_dim).zero_())
self.inputs = inputs
- self.processed_inputs = self.attention_layer.inputs_layer(inputs)
+ self.processed_inputs = self.attention.inputs_layer(inputs)
self.mask = mask
def _reshape_memory(self, memories):
@@ -193,18 +193,18 @@ class Decoder(nn.Module):
return outputs, stop_tokens, alignments
def decode(self, memory):
- cell_input = torch.cat((memory, self.context), -1)
- self.attention_hidden, self.attention_cell = self.attention_rnn(
- cell_input, (self.attention_hidden, self.attention_cell))
- self.attention_hidden = F.dropout(
- self.attention_hidden, self.p_attention_dropout, self.training)
- self.attention_cell = F.dropout(
- self.attention_cell, self.p_attention_dropout, self.training)
+ query_input = torch.cat((memory, self.context), -1)
+ self.query, self.attention_rnn_cell_state = self.attention_rnn(
+ query_input, (self.query, self.attention_rnn_cell_state))
+ self.query = F.dropout(
+ self.query, self.p_attention_dropout, self.training)
+ self.attention_rnn_cell_state = F.dropout(
+ self.attention_rnn_cell_state, self.p_attention_dropout, self.training)
- self.context = self.attention_layer(self.attention_hidden, self.inputs,
- self.processed_inputs, self.mask)
+ self.context = self.attention(self.query, self.inputs,
+ self.processed_inputs, self.mask)
- memory = torch.cat((self.attention_hidden, self.context), -1)
+ memory = torch.cat((self.query, self.context), -1)
self.decoder_hidden, self.decoder_cell = self.decoder_rnn(
memory, (self.decoder_hidden, self.decoder_cell))
self.decoder_hidden = F.dropout(self.decoder_hidden,
@@ -223,7 +223,7 @@ class Decoder(nn.Module):
stop_token = self.stopnet(stopnet_input.detach())
else:
stop_token = self.stopnet(stopnet_input)
- return decoder_output, stop_token, self.attention_layer.attention_weights
+ return decoder_output, stop_token, self.attention.attention_weights
def forward(self, inputs, memories, mask):
memory = self.get_go_frame(inputs).unsqueeze(0)
@@ -232,7 +232,7 @@ class Decoder(nn.Module):
memories = self.prenet(memories)
self._init_states(inputs, mask=mask)
- self.attention_layer.init_states(inputs)
+ self.attention.init_states(inputs)
outputs, stop_tokens, alignments = [], [], []
while len(outputs) < memories.size(0) - 1:
@@ -251,8 +251,8 @@ class Decoder(nn.Module):
memory = self.get_go_frame(inputs)
self._init_states(inputs, mask=None)
- self.attention_layer.init_win_idx()
- self.attention_layer.init_states(inputs)
+ self.attention.init_win_idx()
+ self.attention.init_states(inputs)
outputs, stop_tokens, alignments, t = [], [], [], 0
stop_flags = [True, False, False]
@@ -295,8 +295,8 @@ class Decoder(nn.Module):
else:
self._init_states(inputs, mask=None, keep_states=True)
- self.attention_layer.init_win_idx()
- self.attention_layer.init_states(inputs)
+ self.attention.init_win_idx()
+ self.attention.init_states(inputs)
outputs, stop_tokens, alignments, t = [], [], [], 0
stop_flags = [True, False, False]
stop_count = 0
diff --git a/models/tacotron.py b/models/tacotron.py
index b7f40683..69a6fa03 100644
--- a/models/tacotron.py
+++ b/models/tacotron.py
@@ -1,7 +1,7 @@
# coding: utf-8
from torch import nn
-from layers.tacotron import Encoder, Decoder, PostCBHG
-from utils.generic_utils import sequence_mask
+from TTS.layers.tacotron import Encoder, Decoder, PostCBHG
+from TTS.utils.generic_utils import sequence_mask
class Tacotron(nn.Module):
@@ -36,10 +36,8 @@ class Tacotron(nn.Module):
forward_attn, trans_agent, forward_attn_mask,
location_attn, separate_stopnet)
self.postnet = PostCBHG(mel_dim)
- self.last_linear = nn.Sequential(
- nn.Linear(self.postnet.cbhg.gru_features * 2, linear_dim),
- nn.Sigmoid())
-
+ self.last_linear = nn.Linear(self.postnet.cbhg.gru_features * 2, linear_dim)
+
def forward(self, characters, text_lengths, mel_specs, speaker_ids=None):
B = characters.size(0)
mask = sequence_mask(text_lengths).to(characters.device)
diff --git a/models/tacotron2.py b/models/tacotron2.py
index 05b4c0fd..a91d6e2e 100644
--- a/models/tacotron2.py
+++ b/models/tacotron2.py
@@ -1,7 +1,7 @@
from math import sqrt
from torch import nn
-from layers.tacotron2 import Encoder, Decoder, Postnet
-from utils.generic_utils import sequence_mask
+from TTS.layers.tacotron2 import Encoder, Decoder, Postnet
+from TTS.utils.generic_utils import sequence_mask
# TODO: match function arguments with tacotron
diff --git a/models/tacotrongst.py b/models/tacotrongst.py
index 5b372338..5ea389d9 100644
--- a/models/tacotrongst.py
+++ b/models/tacotrongst.py
@@ -1,8 +1,8 @@
# coding: utf-8
from torch import nn
-from layers.tacotron import Encoder, Decoder, PostCBHG
-from layers.gst_layers import GST
-from utils.generic_utils import sequence_mask
+from TTS.layers.tacotron import Encoder, Decoder, PostCBHG
+from TTS.layers.gst_layers import GST
+from TTS.utils.generic_utils import sequence_mask
class TacotronGST(nn.Module):
@@ -38,9 +38,8 @@ class TacotronGST(nn.Module):
forward_attn, trans_agent, forward_attn_mask,
location_attn, separate_stopnet)
self.postnet = PostCBHG(mel_dim)
- self.last_linear = nn.Sequential(
- nn.Linear(self.postnet.cbhg.gru_features * 2, linear_dim),
- nn.Sigmoid())
+ self.last_linear = nn.Linear(self.postnet.cbhg.gru_features * 2, linear_dim)
+
def forward(self, characters, text_lengths, mel_specs, speaker_ids=None):
B = characters.size(0)
diff --git a/notebooks/Benchmark.ipynb b/notebooks/Benchmark.ipynb
index f06aca80..4de29af9 100644
--- a/notebooks/Benchmark.ipynb
+++ b/notebooks/Benchmark.ipynb
@@ -19,10 +19,8 @@
},
{
"cell_type": "code",
- "execution_count": null,
- "metadata": {
- "collapsed": true
- },
+ "execution_count": 1,
+ "metadata": {},
"outputs": [],
"source": [
"TTS_PATH = \"/home/erogol/projects/\"\n",
@@ -31,12 +29,28 @@
},
{
"cell_type": "code",
- "execution_count": null,
+ "execution_count": 2,
"metadata": {
- "collapsed": true,
"scrolled": true
},
- "outputs": [],
+ "outputs": [
+ {
+ "name": "stdout",
+ "output_type": "stream",
+ "text": [
+ "Populating the interactive namespace from numpy and matplotlib\n"
+ ]
+ },
+ {
+ "name": "stderr",
+ "output_type": "stream",
+ "text": [
+ "/home/erogol/miniconda3/lib/python3.7/site-packages/IPython/core/magics/pylab.py:160: UserWarning: pylab import has clobbered these variables: ['plt']\n",
+ "`%matplotlib` prevents importing * from pylab and numpy\n",
+ " \"\\n`%matplotlib` prevents importing * from pylab and numpy\"\n"
+ ]
+ }
+ ],
"source": [
"%load_ext autoreload\n",
"%autoreload 2\n",
@@ -78,10 +92,8 @@
},
{
"cell_type": "code",
- "execution_count": null,
- "metadata": {
- "collapsed": true
- },
+ "execution_count": 3,
+ "metadata": {},
"outputs": [],
"source": [
"def tts(model, text, CONFIG, use_cuda, ap, use_gl, speaker_id=None, figures=True):\n",
@@ -105,14 +117,25 @@
},
{
"cell_type": "code",
- "execution_count": null,
- "metadata": {
- "collapsed": true
- },
- "outputs": [],
+ "execution_count": 9,
+ "metadata": {},
+ "outputs": [
+ {
+ "ename": "FileNotFoundError",
+ "evalue": "[Errno 2] No such file or directory: '/media/erogol/data_ssd/Data/models/wavernn/mozilla/mozilla-May24-4763/config.json'",
+ "output_type": "error",
+ "traceback": [
+ "\u001b[0;31m---------------------------------------------------------------------------\u001b[0m",
+ "\u001b[0;31mFileNotFoundError\u001b[0m Traceback (most recent call last)",
+ "\u001b[0;32m\u001b[0m in \u001b[0;36m\u001b[0;34m\u001b[0m\n\u001b[1;32m 7\u001b[0m \u001b[0mVOCODER_MODEL_PATH\u001b[0m \u001b[0;34m=\u001b[0m \u001b[0;34m\"/media/erogol/data_ssd/Data/models/wavernn/mozilla/mozilla-May24-4763/model_checkpoints/best_model.pth.tar\"\u001b[0m\u001b[0;34m\u001b[0m\u001b[0;34m\u001b[0m\u001b[0m\n\u001b[1;32m 8\u001b[0m \u001b[0mVOCODER_CONFIG_PATH\u001b[0m \u001b[0;34m=\u001b[0m \u001b[0;34m\"/media/erogol/data_ssd/Data/models/wavernn/mozilla/mozilla-May24-4763/config.json\"\u001b[0m\u001b[0;34m\u001b[0m\u001b[0;34m\u001b[0m\u001b[0m\n\u001b[0;32m----> 9\u001b[0;31m \u001b[0mVOCODER_CONFIG\u001b[0m \u001b[0;34m=\u001b[0m \u001b[0mload_config\u001b[0m\u001b[0;34m(\u001b[0m\u001b[0mVOCODER_CONFIG_PATH\u001b[0m\u001b[0;34m)\u001b[0m\u001b[0;34m\u001b[0m\u001b[0;34m\u001b[0m\u001b[0m\n\u001b[0m\u001b[1;32m 10\u001b[0m \u001b[0muse_cuda\u001b[0m \u001b[0;34m=\u001b[0m \u001b[0;32mFalse\u001b[0m\u001b[0;34m\u001b[0m\u001b[0;34m\u001b[0m\u001b[0m\n\u001b[1;32m 11\u001b[0m \u001b[0;34m\u001b[0m\u001b[0m\n",
+ "\u001b[0;32m~/projects/TTS/tts_namespace/TTS/utils/generic_utils.py\u001b[0m in \u001b[0;36mload_config\u001b[0;34m(config_path)\u001b[0m\n\u001b[1;32m 20\u001b[0m \u001b[0;32mdef\u001b[0m \u001b[0mload_config\u001b[0m\u001b[0;34m(\u001b[0m\u001b[0mconfig_path\u001b[0m\u001b[0;34m)\u001b[0m\u001b[0;34m:\u001b[0m\u001b[0;34m\u001b[0m\u001b[0;34m\u001b[0m\u001b[0m\n\u001b[1;32m 21\u001b[0m \u001b[0mconfig\u001b[0m \u001b[0;34m=\u001b[0m \u001b[0mAttrDict\u001b[0m\u001b[0;34m(\u001b[0m\u001b[0;34m)\u001b[0m\u001b[0;34m\u001b[0m\u001b[0;34m\u001b[0m\u001b[0m\n\u001b[0;32m---> 22\u001b[0;31m \u001b[0;32mwith\u001b[0m \u001b[0mopen\u001b[0m\u001b[0;34m(\u001b[0m\u001b[0mconfig_path\u001b[0m\u001b[0;34m,\u001b[0m \u001b[0;34m\"r\"\u001b[0m\u001b[0;34m)\u001b[0m \u001b[0;32mas\u001b[0m \u001b[0mf\u001b[0m\u001b[0;34m:\u001b[0m\u001b[0;34m\u001b[0m\u001b[0;34m\u001b[0m\u001b[0m\n\u001b[0m\u001b[1;32m 23\u001b[0m \u001b[0minput_str\u001b[0m \u001b[0;34m=\u001b[0m \u001b[0mf\u001b[0m\u001b[0;34m.\u001b[0m\u001b[0mread\u001b[0m\u001b[0;34m(\u001b[0m\u001b[0;34m)\u001b[0m\u001b[0;34m\u001b[0m\u001b[0;34m\u001b[0m\u001b[0m\n\u001b[1;32m 24\u001b[0m \u001b[0minput_str\u001b[0m \u001b[0;34m=\u001b[0m \u001b[0mre\u001b[0m\u001b[0;34m.\u001b[0m\u001b[0msub\u001b[0m\u001b[0;34m(\u001b[0m\u001b[0;34mr'\\\\\\n'\u001b[0m\u001b[0;34m,\u001b[0m \u001b[0;34m''\u001b[0m\u001b[0;34m,\u001b[0m \u001b[0minput_str\u001b[0m\u001b[0;34m)\u001b[0m\u001b[0;34m\u001b[0m\u001b[0;34m\u001b[0m\u001b[0m\n",
+ "\u001b[0;31mFileNotFoundError\u001b[0m: [Errno 2] No such file or directory: '/media/erogol/data_ssd/Data/models/wavernn/mozilla/mozilla-May24-4763/config.json'"
+ ]
+ }
+ ],
"source": [
"# Set constants\n",
- "ROOT_PATH = '/media/erogol/data_ssd/Data/models/mozilla_models/4845/'\n",
+ "ROOT_PATH = '/media/erogol/data_ssd/Models/libri_tts/5049/'\n",
"MODEL_PATH = ROOT_PATH + 'best_model.pth.tar'\n",
"CONFIG_PATH = ROOT_PATH + '/config.json'\n",
"OUT_FOLDER = \"/home/erogol/Dropbox/AudioSamples/benchmark_samples/\"\n",
@@ -136,9 +159,7 @@
{
"cell_type": "code",
"execution_count": null,
- "metadata": {
- "collapsed": true
- },
+ "metadata": {},
"outputs": [],
"source": [
"# LOAD TTS MODEL\n",
@@ -169,9 +190,7 @@
{
"cell_type": "code",
"execution_count": null,
- "metadata": {
- "collapsed": true
- },
+ "metadata": {},
"outputs": [],
"source": [
"# LOAD WAVERNN\n",
@@ -211,12 +230,21 @@
},
{
"cell_type": "code",
- "execution_count": null,
- "metadata": {
- "collapsed": true,
- "scrolled": false
- },
- "outputs": [],
+ "execution_count": 5,
+ "metadata": {},
+ "outputs": [
+ {
+ "ename": "NameError",
+ "evalue": "name 'model' is not defined",
+ "output_type": "error",
+ "traceback": [
+ "\u001b[0;31m---------------------------------------------------------------------------\u001b[0m",
+ "\u001b[0;31mNameError\u001b[0m Traceback (most recent call last)",
+ "\u001b[0;32m\u001b[0m in \u001b[0;36m\u001b[0;34m\u001b[0m\n\u001b[0;32m----> 1\u001b[0;31m \u001b[0mmodel\u001b[0m\u001b[0;34m.\u001b[0m\u001b[0meval\u001b[0m\u001b[0;34m(\u001b[0m\u001b[0;34m)\u001b[0m\u001b[0;34m\u001b[0m\u001b[0;34m\u001b[0m\u001b[0m\n\u001b[0m\u001b[1;32m 2\u001b[0m \u001b[0mmodel\u001b[0m\u001b[0;34m.\u001b[0m\u001b[0mdecoder\u001b[0m\u001b[0;34m.\u001b[0m\u001b[0mmax_decoder_steps\u001b[0m \u001b[0;34m=\u001b[0m \u001b[0;36m2000\u001b[0m\u001b[0;34m\u001b[0m\u001b[0;34m\u001b[0m\u001b[0m\n\u001b[1;32m 3\u001b[0m \u001b[0mspeaker_id\u001b[0m \u001b[0;34m=\u001b[0m \u001b[0;36m0\u001b[0m\u001b[0;34m\u001b[0m\u001b[0;34m\u001b[0m\u001b[0m\n\u001b[1;32m 4\u001b[0m \u001b[0msentence\u001b[0m \u001b[0;34m=\u001b[0m \u001b[0;34m\"Bill got in the habit of asking himself “Is that thought true?” And if he wasn’t absolutely certain it was, he just let it go.\"\u001b[0m\u001b[0;34m\u001b[0m\u001b[0;34m\u001b[0m\u001b[0m\n\u001b[1;32m 5\u001b[0m \u001b[0malign\u001b[0m\u001b[0;34m,\u001b[0m \u001b[0mspec\u001b[0m\u001b[0;34m,\u001b[0m \u001b[0mstop_tokens\u001b[0m\u001b[0;34m,\u001b[0m \u001b[0mwav\u001b[0m \u001b[0;34m=\u001b[0m \u001b[0mtts\u001b[0m\u001b[0;34m(\u001b[0m\u001b[0mmodel\u001b[0m\u001b[0;34m,\u001b[0m \u001b[0msentence\u001b[0m\u001b[0;34m,\u001b[0m \u001b[0mCONFIG\u001b[0m\u001b[0;34m,\u001b[0m \u001b[0muse_cuda\u001b[0m\u001b[0;34m,\u001b[0m \u001b[0map\u001b[0m\u001b[0;34m,\u001b[0m \u001b[0mspeaker_id\u001b[0m\u001b[0;34m=\u001b[0m\u001b[0mspeaker_id\u001b[0m\u001b[0;34m,\u001b[0m \u001b[0muse_gl\u001b[0m\u001b[0;34m=\u001b[0m\u001b[0muse_gl\u001b[0m\u001b[0;34m,\u001b[0m \u001b[0mfigures\u001b[0m\u001b[0;34m=\u001b[0m\u001b[0;32mTrue\u001b[0m\u001b[0;34m)\u001b[0m\u001b[0;34m\u001b[0m\u001b[0;34m\u001b[0m\u001b[0m\n",
+ "\u001b[0;31mNameError\u001b[0m: name 'model' is not defined"
+ ]
+ }
+ ],
"source": [
"model.eval()\n",
"model.decoder.max_decoder_steps = 2000\n",
@@ -227,12 +255,23 @@
},
{
"cell_type": "code",
- "execution_count": null,
+ "execution_count": 6,
"metadata": {
- "collapsed": true,
"scrolled": true
},
- "outputs": [],
+ "outputs": [
+ {
+ "ename": "NameError",
+ "evalue": "name 'model' is not defined",
+ "output_type": "error",
+ "traceback": [
+ "\u001b[0;31m---------------------------------------------------------------------------\u001b[0m",
+ "\u001b[0;31mNameError\u001b[0m Traceback (most recent call last)",
+ "\u001b[0;32m\u001b[0m in \u001b[0;36m\u001b[0;34m\u001b[0m\n\u001b[1;32m 1\u001b[0m \u001b[0msentence\u001b[0m \u001b[0;34m=\u001b[0m \u001b[0;34m\"Be a voice, not an echo.\"\u001b[0m \u001b[0;31m# 'echo' is not in training set.\u001b[0m\u001b[0;34m\u001b[0m\u001b[0;34m\u001b[0m\u001b[0m\n\u001b[0;32m----> 2\u001b[0;31m \u001b[0malign\u001b[0m\u001b[0;34m,\u001b[0m \u001b[0mspec\u001b[0m\u001b[0;34m,\u001b[0m \u001b[0mstop_tokens\u001b[0m\u001b[0;34m,\u001b[0m \u001b[0mwav\u001b[0m \u001b[0;34m=\u001b[0m \u001b[0mtts\u001b[0m\u001b[0;34m(\u001b[0m\u001b[0mmodel\u001b[0m\u001b[0;34m,\u001b[0m \u001b[0msentence\u001b[0m\u001b[0;34m,\u001b[0m \u001b[0mCONFIG\u001b[0m\u001b[0;34m,\u001b[0m \u001b[0muse_cuda\u001b[0m\u001b[0;34m,\u001b[0m \u001b[0map\u001b[0m\u001b[0;34m,\u001b[0m \u001b[0mspeaker_id\u001b[0m\u001b[0;34m=\u001b[0m\u001b[0mspeaker_id\u001b[0m\u001b[0;34m,\u001b[0m \u001b[0muse_gl\u001b[0m\u001b[0;34m=\u001b[0m\u001b[0muse_gl\u001b[0m\u001b[0;34m,\u001b[0m \u001b[0mfigures\u001b[0m\u001b[0;34m=\u001b[0m\u001b[0;32mTrue\u001b[0m\u001b[0;34m)\u001b[0m\u001b[0;34m\u001b[0m\u001b[0;34m\u001b[0m\u001b[0m\n\u001b[0m",
+ "\u001b[0;31mNameError\u001b[0m: name 'model' is not defined"
+ ]
+ }
+ ],
"source": [
"sentence = \"Be a voice, not an echo.\" # 'echo' is not in training set. \n",
"align, spec, stop_tokens, wav = tts(model, sentence, CONFIG, use_cuda, ap, speaker_id=speaker_id, use_gl=use_gl, figures=True)"
@@ -240,11 +279,21 @@
},
{
"cell_type": "code",
- "execution_count": null,
- "metadata": {
- "collapsed": true
- },
- "outputs": [],
+ "execution_count": 7,
+ "metadata": {},
+ "outputs": [
+ {
+ "ename": "NameError",
+ "evalue": "name 'model' is not defined",
+ "output_type": "error",
+ "traceback": [
+ "\u001b[0;31m---------------------------------------------------------------------------\u001b[0m",
+ "\u001b[0;31mNameError\u001b[0m Traceback (most recent call last)",
+ "\u001b[0;32m\u001b[0m in \u001b[0;36m\u001b[0;34m\u001b[0m\n\u001b[1;32m 1\u001b[0m \u001b[0msentence\u001b[0m \u001b[0;34m=\u001b[0m \u001b[0;34m\"The human voice is the most perfect instrument of all.\"\u001b[0m\u001b[0;34m\u001b[0m\u001b[0;34m\u001b[0m\u001b[0m\n\u001b[0;32m----> 2\u001b[0;31m \u001b[0malign\u001b[0m\u001b[0;34m,\u001b[0m \u001b[0mspec\u001b[0m\u001b[0;34m,\u001b[0m \u001b[0mstop_tokens\u001b[0m\u001b[0;34m,\u001b[0m \u001b[0mwav\u001b[0m \u001b[0;34m=\u001b[0m \u001b[0mtts\u001b[0m\u001b[0;34m(\u001b[0m\u001b[0mmodel\u001b[0m\u001b[0;34m,\u001b[0m \u001b[0msentence\u001b[0m\u001b[0;34m,\u001b[0m \u001b[0mCONFIG\u001b[0m\u001b[0;34m,\u001b[0m \u001b[0muse_cuda\u001b[0m\u001b[0;34m,\u001b[0m \u001b[0map\u001b[0m\u001b[0;34m,\u001b[0m \u001b[0mspeaker_id\u001b[0m\u001b[0;34m=\u001b[0m\u001b[0mspeaker_id\u001b[0m\u001b[0;34m,\u001b[0m \u001b[0muse_gl\u001b[0m\u001b[0;34m=\u001b[0m\u001b[0muse_gl\u001b[0m\u001b[0;34m,\u001b[0m \u001b[0mfigures\u001b[0m\u001b[0;34m=\u001b[0m\u001b[0;32mTrue\u001b[0m\u001b[0;34m)\u001b[0m\u001b[0;34m\u001b[0m\u001b[0;34m\u001b[0m\u001b[0m\n\u001b[0m",
+ "\u001b[0;31mNameError\u001b[0m: name 'model' is not defined"
+ ]
+ }
+ ],
"source": [
"sentence = \"The human voice is the most perfect instrument of all.\"\n",
"align, spec, stop_tokens, wav = tts(model, sentence, CONFIG, use_cuda, ap, speaker_id=speaker_id, use_gl=use_gl, figures=True)"
@@ -252,11 +301,21 @@
},
{
"cell_type": "code",
- "execution_count": null,
- "metadata": {
- "collapsed": true
- },
- "outputs": [],
+ "execution_count": 8,
+ "metadata": {},
+ "outputs": [
+ {
+ "ename": "NameError",
+ "evalue": "name 'model' is not defined",
+ "output_type": "error",
+ "traceback": [
+ "\u001b[0;31m---------------------------------------------------------------------------\u001b[0m",
+ "\u001b[0;31mNameError\u001b[0m Traceback (most recent call last)",
+ "\u001b[0;32m\u001b[0m in \u001b[0;36m\u001b[0;34m\u001b[0m\n\u001b[1;32m 1\u001b[0m \u001b[0msentence\u001b[0m \u001b[0;34m=\u001b[0m \u001b[0;34m\"I'm sorry Dave. I'm afraid I can't do that.\"\u001b[0m\u001b[0;34m\u001b[0m\u001b[0;34m\u001b[0m\u001b[0m\n\u001b[0;32m----> 2\u001b[0;31m \u001b[0malign\u001b[0m\u001b[0;34m,\u001b[0m \u001b[0mspec\u001b[0m\u001b[0;34m,\u001b[0m \u001b[0mstop_tokens\u001b[0m\u001b[0;34m,\u001b[0m \u001b[0mwav\u001b[0m \u001b[0;34m=\u001b[0m \u001b[0mtts\u001b[0m\u001b[0;34m(\u001b[0m\u001b[0mmodel\u001b[0m\u001b[0;34m,\u001b[0m \u001b[0msentence\u001b[0m\u001b[0;34m,\u001b[0m \u001b[0mCONFIG\u001b[0m\u001b[0;34m,\u001b[0m \u001b[0muse_cuda\u001b[0m\u001b[0;34m,\u001b[0m \u001b[0map\u001b[0m\u001b[0;34m,\u001b[0m \u001b[0mspeaker_id\u001b[0m\u001b[0;34m=\u001b[0m\u001b[0mspeaker_id\u001b[0m\u001b[0;34m,\u001b[0m \u001b[0muse_gl\u001b[0m\u001b[0;34m=\u001b[0m\u001b[0muse_gl\u001b[0m\u001b[0;34m,\u001b[0m \u001b[0mfigures\u001b[0m\u001b[0;34m=\u001b[0m\u001b[0;32mTrue\u001b[0m\u001b[0;34m)\u001b[0m\u001b[0;34m\u001b[0m\u001b[0;34m\u001b[0m\u001b[0m\n\u001b[0m",
+ "\u001b[0;31mNameError\u001b[0m: name 'model' is not defined"
+ ]
+ }
+ ],
"source": [
"sentence = \"I'm sorry Dave. I'm afraid I can't do that.\"\n",
"align, spec, stop_tokens, wav = tts(model, sentence, CONFIG, use_cuda, ap, speaker_id=speaker_id, use_gl=use_gl, figures=True)"
@@ -267,6 +326,9 @@
"execution_count": null,
"metadata": {
"collapsed": true,
+ "jupyter": {
+ "outputs_hidden": true
+ },
"scrolled": true
},
"outputs": [],
@@ -286,7 +348,10 @@
"cell_type": "code",
"execution_count": null,
"metadata": {
- "collapsed": true
+ "collapsed": true,
+ "jupyter": {
+ "outputs_hidden": true
+ }
},
"outputs": [],
"source": [
@@ -298,7 +363,10 @@
"cell_type": "code",
"execution_count": null,
"metadata": {
- "collapsed": true
+ "collapsed": true,
+ "jupyter": {
+ "outputs_hidden": true
+ }
},
"outputs": [],
"source": [
@@ -310,7 +378,10 @@
"cell_type": "code",
"execution_count": null,
"metadata": {
- "collapsed": true
+ "collapsed": true,
+ "jupyter": {
+ "outputs_hidden": true
+ }
},
"outputs": [],
"source": [
@@ -322,7 +393,10 @@
"cell_type": "code",
"execution_count": null,
"metadata": {
- "collapsed": true
+ "collapsed": true,
+ "jupyter": {
+ "outputs_hidden": true
+ }
},
"outputs": [],
"source": [
@@ -334,7 +408,10 @@
"cell_type": "code",
"execution_count": null,
"metadata": {
- "collapsed": true
+ "collapsed": true,
+ "jupyter": {
+ "outputs_hidden": true
+ }
},
"outputs": [],
"source": [
@@ -353,7 +430,10 @@
"cell_type": "code",
"execution_count": null,
"metadata": {
- "collapsed": true
+ "collapsed": true,
+ "jupyter": {
+ "outputs_hidden": true
+ }
},
"outputs": [],
"source": [
@@ -365,7 +445,10 @@
"cell_type": "code",
"execution_count": null,
"metadata": {
- "collapsed": true
+ "collapsed": true,
+ "jupyter": {
+ "outputs_hidden": true
+ }
},
"outputs": [],
"source": [
@@ -377,7 +460,10 @@
"cell_type": "code",
"execution_count": null,
"metadata": {
- "collapsed": true
+ "collapsed": true,
+ "jupyter": {
+ "outputs_hidden": true
+ }
},
"outputs": [],
"source": [
@@ -389,7 +475,10 @@
"cell_type": "code",
"execution_count": null,
"metadata": {
- "collapsed": true
+ "collapsed": true,
+ "jupyter": {
+ "outputs_hidden": true
+ }
},
"outputs": [],
"source": [
@@ -402,7 +491,9 @@
"execution_count": null,
"metadata": {
"collapsed": true,
- "scrolled": false
+ "jupyter": {
+ "outputs_hidden": true
+ }
},
"outputs": [],
"source": [
@@ -415,7 +506,9 @@
"execution_count": null,
"metadata": {
"collapsed": true,
- "scrolled": false
+ "jupyter": {
+ "outputs_hidden": true
+ }
},
"outputs": [],
"source": [
@@ -427,7 +520,10 @@
"cell_type": "code",
"execution_count": null,
"metadata": {
- "collapsed": true
+ "collapsed": true,
+ "jupyter": {
+ "outputs_hidden": true
+ }
},
"outputs": [],
"source": [
@@ -439,7 +535,10 @@
"cell_type": "code",
"execution_count": null,
"metadata": {
- "collapsed": true
+ "collapsed": true,
+ "jupyter": {
+ "outputs_hidden": true
+ }
},
"outputs": [],
"source": [
@@ -451,7 +550,10 @@
"cell_type": "code",
"execution_count": null,
"metadata": {
- "collapsed": true
+ "collapsed": true,
+ "jupyter": {
+ "outputs_hidden": true
+ }
},
"outputs": [],
"source": [
@@ -462,9 +564,7 @@
{
"cell_type": "code",
"execution_count": null,
- "metadata": {
- "collapsed": true
- },
+ "metadata": {},
"outputs": [],
"source": [
"sentence = \"Eren, how are you?\"\n",
@@ -482,7 +582,10 @@
"cell_type": "code",
"execution_count": null,
"metadata": {
- "collapsed": true
+ "collapsed": true,
+ "jupyter": {
+ "outputs_hidden": true
+ }
},
"outputs": [],
"source": [
@@ -494,7 +597,10 @@
"cell_type": "code",
"execution_count": null,
"metadata": {
- "collapsed": true
+ "collapsed": true,
+ "jupyter": {
+ "outputs_hidden": true
+ }
},
"outputs": [],
"source": [
@@ -506,7 +612,10 @@
"cell_type": "code",
"execution_count": null,
"metadata": {
- "collapsed": true
+ "collapsed": true,
+ "jupyter": {
+ "outputs_hidden": true
+ }
},
"outputs": [],
"source": [
@@ -518,7 +627,10 @@
"cell_type": "code",
"execution_count": null,
"metadata": {
- "collapsed": true
+ "collapsed": true,
+ "jupyter": {
+ "outputs_hidden": true
+ }
},
"outputs": [],
"source": [
@@ -531,6 +643,9 @@
"execution_count": null,
"metadata": {
"collapsed": true,
+ "jupyter": {
+ "outputs_hidden": true
+ },
"scrolled": true
},
"outputs": [],
@@ -543,7 +658,10 @@
"cell_type": "code",
"execution_count": null,
"metadata": {
- "collapsed": true
+ "collapsed": true,
+ "jupyter": {
+ "outputs_hidden": true
+ }
},
"outputs": [],
"source": [
@@ -556,7 +674,10 @@
"cell_type": "code",
"execution_count": null,
"metadata": {
- "collapsed": true
+ "collapsed": true,
+ "jupyter": {
+ "outputs_hidden": true
+ }
},
"outputs": [],
"source": [
@@ -566,9 +687,9 @@
],
"metadata": {
"kernelspec": {
- "display_name": "Python 3(mztts)",
+ "display_name": "Python 3",
"language": "python",
- "name": "mztts"
+ "name": "python3"
},
"language_info": {
"codemirror_mode": {
@@ -580,9 +701,9 @@
"name": "python",
"nbconvert_exporter": "python",
"pygments_lexer": "ipython3",
- "version": "3.6.8"
+ "version": "3.7.3"
}
},
"nbformat": 4,
- "nbformat_minor": 2
+ "nbformat_minor": 4
}
diff --git a/notebooks/ExtractTTSpectrogram.ipynb b/notebooks/ExtractTTSpectrogram.ipynb
index f044300d..a0d0be60 100644
--- a/notebooks/ExtractTTSpectrogram.ipynb
+++ b/notebooks/ExtractTTSpectrogram.ipynb
@@ -105,10 +105,10 @@
"metadata": {},
"outputs": [],
"source": [
- "from utils.text.symbols import symbols, phonemes\n",
- "from utils.generic_utils import sequence_mask\n",
- "from layers.losses import L1LossMasked\n",
- "from utils.text.symbols import symbols, phonemes\n",
+ "from TTS.utils.text.symbols import symbols, phonemes\n",
+ "from TTS.utils.generic_utils import sequence_mask\n",
+ "from TTS.layers.losses import L1LossMasked\n",
+ "from TTS.utils.text.symbols import symbols, phonemes\n",
"\n",
"# load the model\n",
"num_chars = len(phonemes) if C.use_phonemes else len(symbols)\n",
diff --git a/server/__init__.py b/server/__init__.py
new file mode 100644
index 00000000..e69de29b
diff --git a/server/conf.json b/server/conf.json
index 6341596d..c8861cd1 100644
--- a/server/conf.json
+++ b/server/conf.json
@@ -1,12 +1,12 @@
{
- "tts_path":"/media/erogol/data_ssd/Models/libri_tts/ljspeech-July-22-2019_10+45AM-ee706b5/", // tts model root folder
+ "tts_path":"/media/erogol/data_ssd/Models/libri_tts/5049/", // tts model root folder
"tts_file":"best_model.pth.tar", // tts checkpoint file
"tts_config":"config.json", // tts config.json file
"tts_speakers": null, // json file listing speaker ids. null if no speaker embedding.
- "wavernn_lib_path": "/home/erogol/projects/", // Rootpath to wavernn project folder to be imported. If this is null, model uses GL for speech synthesis.
- "wavernn_path":"/media/erogol/data_ssd/Models/wavernn/universal/4910/", // wavernn model root path
- "wavernn_file":"best_model_16K.pth.tar", // wavernn checkpoint file name
- "wavernn_config":"config_16K.json", // wavernn config file
+ "wavernn_lib_path": null, // Rootpath to wavernn project folder to be imported. If this is null, model uses GL for speech synthesis.
+ "wavernn_path":null, // wavernn model root path
+ "wavernn_file":null, // wavernn checkpoint file name
+ "wavernn_config": null, // wavernn config file
"is_wavernn_batched":true,
"port": 5002,
"use_cuda": true,
diff --git a/server/server.py b/server/server.py
index 95fa1caf..0244d612 100644
--- a/server/server.py
+++ b/server/server.py
@@ -1,7 +1,7 @@
#!flask/bin/python
import argparse
from synthesizer import Synthesizer
-from utils.generic_utils import load_config
+from TTS.utils.generic_utils import load_config
from flask import Flask, request, render_template, send_file
parser = argparse.ArgumentParser()
diff --git a/server/synthesizer.py b/server/synthesizer.py
index bdfd8c6c..00311914 100644
--- a/server/synthesizer.py
+++ b/server/synthesizer.py
@@ -5,10 +5,11 @@ import numpy as np
import torch
import sys
-from utils.audio import AudioProcessor
-from utils.generic_utils import load_config, setup_model
-from utils.text import phoneme_to_sequence, phonemes, symbols, text_to_sequence, sequence_to_phoneme
-from utils.speakers import load_speaker_mapping
+from TTS.utils.audio import AudioProcessor
+from TTS.utils.generic_utils import load_config, setup_model
+from TTS.utils.text import phonemes, symbols
+from TTS.utils.speakers import load_speaker_mapping
+from TTS.utils.synthesis import *
import re
alphabets = r"([A-Za-z])"
@@ -41,28 +42,25 @@ class Synthesizer(object):
self.ap = AudioProcessor(**self.tts_config.audio)
if self.use_phonemes:
self.input_size = len(phonemes)
- self.input_adapter = lambda sen: phoneme_to_sequence(sen, [self.tts_config.text_cleaner], self.tts_config.phoneme_language, self.tts_config.enable_eos_bos_chars)
else:
self.input_size = len(symbols)
- self.input_adapter = lambda sen: text_to_sequence(sen, [self.tts_config.text_cleaner])
# load speakers
if self.config.tts_speakers is not None:
self.tts_speakers = load_speaker_mapping(os.path.join(model_path, self.config.tts_speakers))
num_speakers = len(self.tts_speakers)
else:
num_speakers = 0
- self.tts_model = setup_model(self.input_size, num_speakers=num_speakers , c=self.tts_config)
+ self.tts_model = setup_model(self.input_size, num_speakers=num_speakers, c=self.tts_config)
# load model state
- if use_cuda:
- cp = torch.load(self.model_file)
- else:
- cp = torch.load(self.model_file, map_location=lambda storage, loc: storage)
+ cp = torch.load(self.model_file)
# load the model
self.tts_model.load_state_dict(cp['model'])
if use_cuda:
self.tts_model.cuda()
self.tts_model.eval()
self.tts_model.decoder.max_decoder_steps = 3000
+ if 'r' in cp and self.tts_config.model in ["Tacotron", "TacotronGST"]:
+ self.tts_model.decoder.set_r(cp['r'])
def load_wavernn(self, lib_path, model_path, model_file, model_config, use_cuda):
# TODO: set a function in wavernn code base for model setup and call it here.
@@ -136,32 +134,27 @@ class Synthesizer(object):
def tts(self, text):
wavs = []
sens = self.split_into_sentences(text)
+ print(sens)
if not sens:
sens = [text+'.']
for sen in sens:
- if len(sen) < 3:
- continue
- sen = sen.strip()
- print(sen)
+ # preprocess the given text
+ inputs = text_to_seqvec(sen, self.tts_config, self.use_cuda)
+ # synthesize voice
+ decoder_output, postnet_output, alignments, _ = run_model(
+ self.tts_model, inputs, self.tts_config, False, None, None)
+ # convert outputs to numpy
+ postnet_output, decoder_output, _ = parse_outputs(
+ postnet_output, decoder_output, alignments)
- seq = np.array(self.input_adapter(sen))
- text_hat = sequence_to_phoneme(seq)
- print(text_hat)
+ if self.wavernn:
+ postnet_output = postnet_output[0].data.cpu().numpy()
+ wav = self.wavernn.generate(torch.FloatTensor(postnet_output.T).unsqueeze(0).cuda(), batched=self.config.is_wavernn_batched, target=11000, overlap=550)
+ else:
+ wav = inv_spectrogram(postnet_output, self.ap, self.tts_config)
+ # trim silence
+ wav = trim_silence(wav, self.ap)
- chars_var = torch.from_numpy(seq).unsqueeze(0).long()
-
- if self.use_cuda:
- chars_var = chars_var.cuda()
- decoder_out, postnet_out, alignments, stop_tokens = self.tts_model.inference(
- chars_var)
- postnet_out = postnet_out[0].data.cpu().numpy()
- if self.tts_config.model == "Tacotron":
- wav = self.ap.inv_spectrogram(postnet_out.T)
- elif self.tts_config.model == "Tacotron2":
- if self.wavernn:
- wav = self.wavernn.generate(torch.FloatTensor(postnet_out.T).unsqueeze(0).cuda(), batched=self.config.is_wavernn_batched, target=11000, overlap=550)
- else:
- wav = self.ap.inv_mel_spectrogram(postnet_out.T)
wavs += list(wav)
wavs += [0] * 10000
diff --git a/setup.py b/setup.py
index b1c4c7ac..f6916741 100644
--- a/setup.py
+++ b/setup.py
@@ -62,7 +62,15 @@ setup(
version=version,
url='https://github.com/mozilla/TTS',
description='Text to Speech with Deep Learning',
- packages=find_packages(),
+ license='MPL-2.0',
+ package_dir={'': 'tts_namespace'},
+ packages=find_packages('tts_namespace'),
+ project_urls={
+ 'Documentation': 'https://github.com/mozilla/TTS/wiki',
+ 'Tracker': 'https://github.com/mozilla/TTS/issues',
+ 'Repository': 'https://github.com/mozilla/TTS',
+ 'Discussions': 'https://discourse.mozilla.org/c/tts',
+ },
cmdclass={
'build_py': build_py,
'develop': develop,
@@ -79,14 +87,10 @@ setup(
"flask",
# "lws",
"tqdm",
- "phonemizer",
"soundfile",
+ "phonemizer @ https://github.com/bootphon/phonemizer/tarball/master",
],
dependency_links=[
- 'http://github.com/bootphon/phonemizer/tarball/master#egg=phonemizer'
- ],
- extras_require={
- "bin": [
- "requests",
- ],
- })
+ "http://github.com/bootphon/phonemizer/tarball/master#egg=phonemizer-1.0.1"
+ ]
+)
diff --git a/synthesize.py b/synthesize.py
index 33a31c69..23c67c73 100644
--- a/synthesize.py
+++ b/synthesize.py
@@ -4,10 +4,10 @@ import argparse
import torch
import string
-from utils.synthesis import synthesis
-from utils.generic_utils import load_config, setup_model
-from utils.text.symbols import symbols, phonemes
-from utils.audio import AudioProcessor
+from TTS.utils.synthesis import synthesis
+from TTS.utils.generic_utils import load_config, setup_model
+from TTS.utils.text.symbols import symbols, phonemes
+from TTS.utils.audio import AudioProcessor
def tts(model,
diff --git a/tests/generic_utils_text.py b/tests/generic_utils_text.py
index 2ef39c09..228df2df 100644
--- a/tests/generic_utils_text.py
+++ b/tests/generic_utils_text.py
@@ -1,8 +1,8 @@
import unittest
import torch as T
-from utils.generic_utils import save_checkpoint, save_best_model
-from layers.tacotron import Prenet
+from TTS.utils.generic_utils import save_checkpoint, save_best_model
+from TTS.layers.tacotron import Prenet
OUT_PATH = '/tmp/test.pth.tar'
diff --git a/tests/inputs/server_config.json b/tests/inputs/server_config.json
index d3220d7d..8ac266bd 100644
--- a/tests/inputs/server_config.json
+++ b/tests/inputs/server_config.json
@@ -1,5 +1,5 @@
{
- "tts_path":"tests/outputs/", // tts model root folder
+ "tts_path":"TTS/tests/outputs/", // tts model root folder
"tts_file":"checkpoint_10.pth.tar", // tts checkpoint file
"tts_config":"dummy_model_config.json", // tts config.json file
"tts_speakers": null, // json file listing speaker ids. null if no speaker embedding.
diff --git a/tests/symbols_tests.py b/tests/symbols_tests.py
index 68c909c5..4c32c7d6 100644
--- a/tests/symbols_tests.py
+++ b/tests/symbols_tests.py
@@ -1,7 +1,8 @@
import unittest
-from utils.text import phonemes
+from TTS.utils.text import phonemes
class SymbolsTest(unittest.TestCase):
- def test_uniqueness(self):
- assert sorted(phonemes) == sorted(list(set(phonemes)))
+ def test_uniqueness(self): #pylint: disable=no-self-use
+ assert sorted(phonemes) == sorted(list(set(phonemes))), " {} vs {} ".format(len(phonemes), len(set(phonemes)))
+
\ No newline at end of file
diff --git a/tests/test_audio.py b/tests/test_audio.py
index b2c4a135..fc5deb48 100644
--- a/tests/test_audio.py
+++ b/tests/test_audio.py
@@ -1,9 +1,9 @@
import os
import unittest
-from tests import get_tests_path, get_tests_input_path, get_tests_output_path
-from utils.audio import AudioProcessor
-from utils.generic_utils import load_config
+from TTS.tests import get_tests_path, get_tests_input_path, get_tests_output_path
+from TTS.utils.audio import AudioProcessor
+from TTS.utils.generic_utils import load_config
TESTS_PATH = get_tests_path()
OUT_PATH = os.path.join(get_tests_output_path(), "audio_tests")
diff --git a/tests/test_demo_server.py b/tests/test_demo_server.py
index 0d0a3ac6..5eb3c01c 100644
--- a/tests/test_demo_server.py
+++ b/tests/test_demo_server.py
@@ -3,10 +3,10 @@ import unittest
import torch as T
-from server.synthesizer import Synthesizer
-from tests import get_tests_input_path, get_tests_output_path, get_tests_path
-from utils.text.symbols import phonemes, symbols
-from utils.generic_utils import load_config, save_checkpoint, setup_model
+from TTS.server.synthesizer import Synthesizer
+from TTS.tests import get_tests_input_path, get_tests_output_path
+from TTS.utils.text.symbols import phonemes, symbols
+from TTS.utils.generic_utils import load_config, save_checkpoint, setup_model
class DemoServerTest(unittest.TestCase):
@@ -20,5 +20,6 @@ class DemoServerTest(unittest.TestCase):
def test_in_out(self):
self._create_random_model()
config = load_config(os.path.join(get_tests_input_path(), 'server_config.json'))
+ config['tts_path'] = get_tests_output_path()
synthesizer = Synthesizer(config)
synthesizer.tts("Better this test works!!")
diff --git a/tests/test_layers.py b/tests/test_layers.py
index 7d9e0650..cf27e30c 100644
--- a/tests/test_layers.py
+++ b/tests/test_layers.py
@@ -1,9 +1,9 @@
import unittest
import torch as T
-from layers.tacotron import Prenet, CBHG, Decoder, Encoder
-from layers.losses import L1LossMasked
-from utils.generic_utils import sequence_mask
+from TTS.layers.tacotron import Prenet, CBHG, Decoder, Encoder
+from TTS.layers.losses import L1LossMasked
+from TTS.utils.generic_utils import sequence_mask
#pylint: disable=unused-variable
diff --git a/tests/test_loader.py b/tests/test_loader.py
index 92d6f7e2..fe1cefef 100644
--- a/tests/test_loader.py
+++ b/tests/test_loader.py
@@ -1,12 +1,14 @@
import os
import unittest
import shutil
+import torch
+import numpy as np
from torch.utils.data import DataLoader
-from utils.generic_utils import load_config
-from utils.audio import AudioProcessor
-from datasets import TTSDataset
-from datasets.preprocess import ljspeech
+from TTS.utils.generic_utils import load_config
+from TTS.utils.audio import AudioProcessor
+from TTS.datasets import TTSDataset
+from TTS.datasets.preprocess import ljspeech
#pylint: disable=unused-variable
@@ -128,12 +130,16 @@ class TestTTSDataset(unittest.TestCase):
item_idx = data[7]
# check mel_spec consistency
- wav = self.ap.load_wav(item_idx[0])
- mel = self.ap.melspectrogram(wav)
- mel_dl = mel_input[0].cpu().numpy()
- assert (abs(mel.T).astype("float32")
- - abs(mel_dl[:-1])
- ).sum() == 0
+ wav = np.asarray(self.ap.load_wav(item_idx[0]), dtype=np.float32)
+ mel = self.ap.melspectrogram(wav).astype('float32')
+ mel = torch.FloatTensor(mel).contiguous()
+ mel_dl = mel_input[0]
+ # NOTE: Below needs to check == 0 but due to an unknown reason
+ # there is a slight difference between two matrices.
+ # TODO: Check this assert cond more in detail.
+ assert abs((abs(mel.T)
+ - abs(mel_dl[:-1])
+ ).sum()) < 1e-5, (abs(mel.T) - abs(mel_dl[:-1])).sum()
# check mel-spec correctness
mel_spec = mel_input[0].cpu().numpy()
diff --git a/tests/test_preprocessors.py b/tests/test_preprocessors.py
index 6f4b6df1..993ee495 100644
--- a/tests/test_preprocessors.py
+++ b/tests/test_preprocessors.py
@@ -1,8 +1,8 @@
import unittest
import os
-from tests import get_tests_input_path
+from TTS.tests import get_tests_input_path
-from datasets.preprocess import common_voice
+from TTS.datasets.preprocess import common_voice
class TestPreprocessors(unittest.TestCase):
diff --git a/tests/test_tacotron2_model.py b/tests/test_tacotron2_model.py
index 9ec2d4dc..a26f1ddf 100644
--- a/tests/test_tacotron2_model.py
+++ b/tests/test_tacotron2_model.py
@@ -6,9 +6,9 @@ import numpy as np
from torch import optim
from torch import nn
-from utils.generic_utils import load_config
-from layers.losses import MSELossMasked
-from models.tacotron2 import Tacotron2
+from TTS.utils.generic_utils import load_config
+from TTS.layers.losses import MSELossMasked
+from TTS.models.tacotron2 import Tacotron2
#pylint: disable=unused-variable
diff --git a/tests/test_tacotron_model.py b/tests/test_tacotron_model.py
index b44cb58f..acd7af41 100644
--- a/tests/test_tacotron_model.py
+++ b/tests/test_tacotron_model.py
@@ -5,9 +5,9 @@ import unittest
from torch import optim
from torch import nn
-from utils.generic_utils import load_config
-from layers.losses import L1LossMasked
-from models.tacotron import Tacotron
+from TTS.utils.generic_utils import load_config
+from TTS.layers.losses import L1LossMasked
+from TTS.models.tacotron import Tacotron
#pylint: disable=unused-variable
diff --git a/tests/test_text_processing.py b/tests/test_text_processing.py
index 62440e47..8f8e6fab 100644
--- a/tests/test_text_processing.py
+++ b/tests/test_text_processing.py
@@ -1,7 +1,7 @@
import unittest
import torch as T
-from utils.text import *
+from TTS.utils.text import *
def test_phoneme_to_sequence():
text = "Recent research at Harvard has shown meditating for as little as 8 weeks can actually increase, the grey matter in the parts of the brain responsible for emotional regulation and learning!"
diff --git a/train.py b/train.py
index 815a0a32..30133b96 100644
--- a/train.py
+++ b/train.py
@@ -10,24 +10,26 @@ import torch.nn as nn
from torch import optim
from torch.utils.data import DataLoader
-from datasets.TTSDataset import MyDataset
+from TTS.datasets.TTSDataset import MyDataset
from distribute import (DistributedSampler, apply_gradient_allreduce,
init_distributed, reduce_tensor)
-from layers.losses import L1LossMasked, MSELossMasked
-from utils.audio import AudioProcessor
-from utils.generic_utils import (NoamLR, check_update, count_parameters,
- create_experiment_folder, get_git_branch,
- load_config, remove_experiment_folder,
- save_best_model, save_checkpoint, weight_decay,
- set_init_dict, copy_config_file, setup_model,
- split_dataset)
-from utils.logger import Logger
-from utils.speakers import load_speaker_mapping, save_speaker_mapping, \
+from TTS.layers.losses import L1LossMasked, MSELossMasked
+from TTS.utils.audio import AudioProcessor
+from TTS.utils.generic_utils import (NoamLR, check_update, count_parameters,
+ create_experiment_folder, get_git_branch,
+ load_config, remove_experiment_folder,
+ save_best_model, save_checkpoint, weight_decay,
+ set_init_dict, copy_config_file, setup_model,
+ split_dataset, gradual_training_scheduler)
+from TTS.utils.logger import Logger
+from TTS.utils.speakers import load_speaker_mapping, save_speaker_mapping, \
get_speakers
-from utils.synthesis import synthesis
-from utils.text.symbols import phonemes, symbols
-from utils.visual import plot_alignment, plot_spectrogram
-from datasets.preprocess import get_preprocessor_by_name
+from TTS.utils.synthesis import synthesis
+from TTS.utils.text.symbols import phonemes, symbols
+from TTS.utils.visual import plot_alignment, plot_spectrogram
+from TTS.datasets.preprocess import get_preprocessor_by_name
+from TTS.utils.radam import RAdam
+
torch.backends.cudnn.enabled = True
torch.backends.cudnn.benchmark = False
@@ -82,7 +84,7 @@ def setup_loader(ap, is_val=False, verbose=False):
def train(model, criterion, criterion_st, optimizer, optimizer_st, scheduler,
- ap, epoch):
+ ap, global_step, epoch):
data_loader = setup_loader(ap, is_val=False, verbose=(epoch == 0))
if c.use_speaker_embedding:
speaker_mapping = load_speaker_mapping(OUT_PATH)
@@ -92,8 +94,13 @@ def train(model, criterion, criterion_st, optimizer, optimizer_st, scheduler,
avg_decoder_loss = 0
avg_stop_loss = 0
avg_step_time = 0
+ avg_loader_time = 0
print("\n > Epoch {}/{}".format(epoch, c.epochs), flush=True)
- batch_n_iter = int(len(data_loader.dataset) / (c.batch_size * num_gpus))
+ if use_cuda:
+ batch_n_iter = int(len(data_loader.dataset) / (c.batch_size * num_gpus))
+ else:
+ batch_n_iter = int(len(data_loader.dataset) / c.batch_size)
+ end_time = time.time()
for num_iter, data in enumerate(data_loader):
start_time = time.time()
@@ -107,6 +114,7 @@ def train(model, criterion, criterion_st, optimizer, optimizer_st, scheduler,
stop_targets = data[6]
avg_text_length = torch.mean(text_lengths.float())
avg_spec_length = torch.mean(mel_lengths.float())
+ loader_time = time.time() - end_time
if c.use_speaker_embedding:
speaker_ids = [speaker_mapping[speaker_name]
@@ -120,8 +128,7 @@ def train(model, criterion, criterion_st, optimizer, optimizer_st, scheduler,
stop_targets.size(1) // c.r, -1)
stop_targets = (stop_targets.sum(2) > 0.0).unsqueeze(2).float().squeeze(2)
- current_step = num_iter + args.restore_step + \
- epoch * len(data_loader) + 1
+ global_step += 1
# setup lr
if c.lr_decay:
@@ -176,18 +183,20 @@ def train(model, criterion, criterion_st, optimizer, optimizer_st, scheduler,
optimizer_st.step()
else:
grad_norm_st = 0
-
+
step_time = time.time() - start_time
epoch_time += step_time
- if current_step % c.print_step == 0:
+ if global_step % c.print_step == 0:
print(
" | > Step:{}/{} GlobalStep:{} TotalLoss:{:.5f} PostnetLoss:{:.5f} "
"DecoderLoss:{:.5f} StopLoss:{:.5f} GradNorm:{:.5f} "
- "GradNormST:{:.5f} AvgTextLen:{:.1f} AvgSpecLen:{:.1f} StepTime:{:.2f} LR:{:.6f}".format(
- num_iter, batch_n_iter, current_step, loss.item(),
+ "GradNormST:{:.5f} AvgTextLen:{:.1f} AvgSpecLen:{:.1f} StepTime:{:.2f} "
+ "LoaderTime:{:.2f} LR:{:.6f}".format(
+ num_iter, batch_n_iter, global_step, loss.item(),
postnet_loss.item(), decoder_loss.item(), stop_loss.item(),
- grad_norm, grad_norm_st, avg_text_length, avg_spec_length, step_time, current_lr),
+ grad_norm, grad_norm_st, avg_text_length, avg_spec_length, step_time,
+ loader_time, current_lr),
flush=True)
# aggregate losses from processes
@@ -202,21 +211,24 @@ def train(model, criterion, criterion_st, optimizer, optimizer_st, scheduler,
avg_decoder_loss += float(decoder_loss.item())
avg_stop_loss += stop_loss if isinstance(stop_loss, float) else float(stop_loss.item())
avg_step_time += step_time
+ avg_loader_time += loader_time
# Plot Training Iter Stats
- iter_stats = {"loss_posnet": postnet_loss.item(),
- "loss_decoder": decoder_loss.item(),
- "lr": current_lr,
- "grad_norm": grad_norm,
- "grad_norm_st": grad_norm_st,
- "step_time": step_time}
- tb_logger.tb_train_iter_stats(current_step, iter_stats)
+ # reduce TB load
+ if global_step % 10 == 0:
+ iter_stats = {"loss_posnet": postnet_loss.item(),
+ "loss_decoder": decoder_loss.item(),
+ "lr": current_lr,
+ "grad_norm": grad_norm,
+ "grad_norm_st": grad_norm_st,
+ "step_time": step_time}
+ tb_logger.tb_train_iter_stats(global_step, iter_stats)
- if current_step % c.save_step == 0:
+ if global_step % c.save_step == 0:
if c.checkpoint:
# save model
save_checkpoint(model, optimizer, optimizer_st,
- postnet_loss.item(), OUT_PATH, current_step,
+ postnet_loss.item(), OUT_PATH, global_step,
epoch)
# Diagnostic visualizations
@@ -229,31 +241,34 @@ def train(model, criterion, criterion_st, optimizer, optimizer_st, scheduler,
"ground_truth": plot_spectrogram(gt_spec, ap),
"alignment": plot_alignment(align_img)
}
- tb_logger.tb_train_figures(current_step, figures)
+ tb_logger.tb_train_figures(global_step, figures)
# Sample audio
if c.model in ["Tacotron", "TacotronGST"]:
train_audio = ap.inv_spectrogram(const_spec.T)
else:
train_audio = ap.inv_mel_spectrogram(const_spec.T)
- tb_logger.tb_train_audios(current_step,
+ tb_logger.tb_train_audios(global_step,
{'TrainAudio': train_audio},
c.audio["sample_rate"])
+ end_time = time.time()
avg_postnet_loss /= (num_iter + 1)
avg_decoder_loss /= (num_iter + 1)
avg_stop_loss /= (num_iter + 1)
avg_total_loss = avg_decoder_loss + avg_postnet_loss + avg_stop_loss
avg_step_time /= (num_iter + 1)
+ avg_loader_time /= (num_iter + 1)
# print epoch stats
print(
" | > EPOCH END -- GlobalStep:{} AvgTotalLoss:{:.5f} "
"AvgPostnetLoss:{:.5f} AvgDecoderLoss:{:.5f} "
"AvgStopLoss:{:.5f} EpochTime:{:.2f} "
- "AvgStepTime:{:.2f}".format(current_step, avg_total_loss,
- avg_postnet_loss, avg_decoder_loss,
- avg_stop_loss, epoch_time, avg_step_time),
+ "AvgStepTime:{:.2f} AvgLoaderTime:{:.2f}".format(global_step, avg_total_loss,
+ avg_postnet_loss, avg_decoder_loss,
+ avg_stop_loss, epoch_time, avg_step_time,
+ avg_loader_time),
flush=True)
# Plot Epoch Stats
@@ -263,14 +278,13 @@ def train(model, criterion, criterion_st, optimizer, optimizer_st, scheduler,
"loss_decoder": avg_decoder_loss,
"stop_loss": avg_stop_loss,
"epoch_time": epoch_time}
- tb_logger.tb_train_epoch_stats(current_step, epoch_stats)
+ tb_logger.tb_train_epoch_stats(global_step, epoch_stats)
if c.tb_model_param_stats:
- tb_logger.tb_model_weights(model, current_step)
-
- return avg_postnet_loss, current_step
+ tb_logger.tb_model_weights(model, global_step)
+ return avg_postnet_loss, global_step
-def evaluate(model, criterion, criterion_st, ap, current_step, epoch):
+def evaluate(model, criterion, criterion_st, ap, global_step, epoch):
data_loader = setup_loader(ap, is_val=True)
if c.use_speaker_embedding:
speaker_mapping = load_speaker_mapping(OUT_PATH)
@@ -383,14 +397,14 @@ def evaluate(model, criterion, criterion_st, ap, current_step, epoch):
"ground_truth": plot_spectrogram(gt_spec, ap),
"alignment": plot_alignment(align_img)
}
- tb_logger.tb_eval_figures(current_step, eval_figures)
+ tb_logger.tb_eval_figures(global_step, eval_figures)
# Sample audio
if c.model in ["Tacotron", "TacotronGST"]:
eval_audio = ap.inv_spectrogram(const_spec.T)
else:
eval_audio = ap.inv_mel_spectrogram(const_spec.T)
- tb_logger.tb_eval_audios(current_step, {"ValAudio": eval_audio}, c.audio["sample_rate"])
+ tb_logger.tb_eval_audios(global_step, {"ValAudio": eval_audio}, c.audio["sample_rate"])
# compute average losses
avg_postnet_loss /= (num_iter + 1)
@@ -401,7 +415,7 @@ def evaluate(model, criterion, criterion_st, ap, current_step, epoch):
epoch_stats = {"loss_postnet": avg_postnet_loss,
"loss_decoder": avg_decoder_loss,
"stop_loss": avg_stop_loss}
- tb_logger.tb_eval_stats(current_step, epoch_stats)
+ tb_logger.tb_eval_stats(global_step, epoch_stats)
if args.rank == 0 and epoch > c.test_delay_epochs:
# test sentences
@@ -409,12 +423,14 @@ def evaluate(model, criterion, criterion_st, ap, current_step, epoch):
test_figures = {}
print(" | > Synthesizing test sentences")
speaker_id = 0 if c.use_speaker_embedding else None
+ style_wav = c.get("style_wav_for_test")
for idx, test_sentence in enumerate(test_sentences):
try:
wav, alignment, decoder_output, postnet_output, stop_tokens = synthesis(
model, test_sentence, c, use_cuda, ap,
- speaker_id=speaker_id)
- file_path = os.path.join(AUDIO_PATH, str(current_step))
+ speaker_id=speaker_id,
+ style_wav=style_wav)
+ file_path = os.path.join(AUDIO_PATH, str(global_step))
os.makedirs(file_path, exist_ok=True)
file_path = os.path.join(file_path,
"TestSentence_{}.wav".format(idx))
@@ -425,8 +441,8 @@ def evaluate(model, criterion, criterion_st, ap, current_step, epoch):
except:
print(" !! Error creating Test Sentence -", idx)
traceback.print_exc()
- tb_logger.tb_test_audios(current_step, test_audios, c.audio['sample_rate'])
- tb_logger.tb_test_figures(current_step, test_figures)
+ tb_logger.tb_test_audios(global_step, test_audios, c.audio['sample_rate'])
+ tb_logger.tb_test_figures(global_step, test_figures)
return avg_postnet_loss
@@ -464,9 +480,9 @@ def main(args): #pylint: disable=redefined-outer-name
print(" | > Num output units : {}".format(ap.num_freq), flush=True)
- optimizer = optim.Adam(model.parameters(), lr=c.lr, weight_decay=0)
+ optimizer = RAdam(model.parameters(), lr=c.lr, weight_decay=0)
if c.stopnet and c.separate_stopnet:
- optimizer_st = optim.Adam(
+ optimizer_st = RAdam(
model.decoder.stopnet.parameters(), lr=c.lr, weight_decay=0)
else:
optimizer_st = None
@@ -524,11 +540,19 @@ def main(args): #pylint: disable=redefined-outer-name
if 'best_loss' not in locals():
best_loss = float('inf')
+ global_step = args.restore_step
for epoch in range(0, c.epochs):
- train_loss, current_step = train(model, criterion, criterion_st,
- optimizer, optimizer_st, scheduler,
- ap, epoch)
- val_loss = evaluate(model, criterion, criterion_st, ap, current_step, epoch)
+ # set gradual training
+ if c.gradual_training is not None:
+ r, c.batch_size = gradual_training_scheduler(global_step, c)
+ c.r = r
+ model.decoder.set_r(r)
+ print(" > Number of outputs per iteration:", model.decoder.r)
+
+ train_loss, global_step = train(model, criterion, criterion_st,
+ optimizer, optimizer_st, scheduler,
+ ap, global_step, epoch)
+ val_loss = evaluate(model, criterion, criterion_st, ap, global_step, epoch)
print(
" | > Training Loss: {:.5f} Validation Loss: {:.5f}".format(
train_loss, val_loss),
@@ -537,7 +561,7 @@ def main(args): #pylint: disable=redefined-outer-name
if c.run_eval:
target_loss = val_loss
best_loss = save_best_model(model, optimizer, target_loss, best_loss,
- OUT_PATH, current_step, epoch)
+ OUT_PATH, global_step, epoch)
if __name__ == '__main__':
@@ -571,7 +595,7 @@ if __name__ == '__main__':
'--output_folder',
type=str,
default='',
- help='folder name for traning outputs.'
+ help='folder name for training outputs.'
)
# DISTRUBUTED
diff --git a/tts_namespace/README.md b/tts_namespace/README.md
new file mode 100644
index 00000000..c5b2ddbf
--- /dev/null
+++ b/tts_namespace/README.md
@@ -0,0 +1,29 @@
+This folder contains a symlink called TTS to the parent folder:
+
+ lrwxr-xr-x TTS -> ..
+
+This is used to appease the distribute/setuptools gods. When the project was
+initially set up, the repository folder itself was considered a namespace, and
+development was done with `sys.path` hacks. This means if you tried to install
+TTS, `setup.py` would see the packages `models`, `utils`, `layers`... instead of
+ `TTS.models`, `TTS.utils`...
+
+Installing TTS would then pollute the package namespace with generic names like
+those above. In order to make things installable in both install and development
+modes (`pip install /path/to/TTS` and `pip install -e /path/to/TTS`), we needed
+to add an additional 'TTS' namespace to avoid this pollution. A virtual redirect
+using `packages_dir` in `setup.py` is not enough because it breaks the editable
+installation, which can only handle the simplest of `package_dir` redirects.
+
+Our solution is to use a symlink in order to add the extra `TTS` namespace. In
+`setup.py`, we only look for packages inside `tts_namespace` (this folder),
+which contains a symlink called TTS pointing to the repository root. The final
+result is that `setuptools.find_packages` will find `TTS.models`, `TTS.utils`...
+
+With this hack, `pip install -e` will then add a symlink to the `tts_namespace`
+in your `site-packages` folder, which works properly. It's important not to add
+anything else in this folder because it will pollute the package namespace when
+installing the project.
+
+This does not work if you check out your project on a filesystem that does not
+support symlinks.
\ No newline at end of file
diff --git a/tts_namespace/TTS b/tts_namespace/TTS
new file mode 120000
index 00000000..a96aa0ea
--- /dev/null
+++ b/tts_namespace/TTS
@@ -0,0 +1 @@
+..
\ No newline at end of file
diff --git a/utils/audio.py b/utils/audio.py
index d4d9d67f..794520af 100644
--- a/utils/audio.py
+++ b/utils/audio.py
@@ -113,8 +113,10 @@ class AudioProcessor(object):
def _stft_parameters(self, ):
"""Compute necessary stft parameters with given time values"""
n_fft = (self.num_freq - 1) * 2
+ factor = self.frame_length_ms / self.frame_shift_ms
+ assert (factor).is_integer(), " [!] frame_shift_ms should divide frame_length_ms"
hop_length = int(self.frame_shift_ms / 1000.0 * self.sample_rate)
- win_length = int(self.frame_length_ms / 1000.0 * self.sample_rate)
+ win_length = int(hop_length * factor)
return n_fft, hop_length, win_length
def _amp_to_db(self, x):
diff --git a/utils/generic_utils.py b/utils/generic_utils.py
index 6cf4f420..1c16834a 100644
--- a/utils/generic_utils.py
+++ b/utils/generic_utils.py
@@ -121,7 +121,8 @@ def save_checkpoint(model, optimizer, optimizer_st, model_loss, out_path,
'step': current_step,
'epoch': epoch,
'linear_loss': model_loss,
- 'date': datetime.date.today().strftime("%B %d, %Y")
+ 'date': datetime.date.today().strftime("%B %d, %Y"),
+ 'r': model.decoder.r
}
torch.save(state, checkpoint_path)
@@ -136,7 +137,8 @@ def save_best_model(model, optimizer, model_loss, best_loss, out_path,
'step': current_step,
'epoch': epoch,
'linear_loss': model_loss,
- 'date': datetime.date.today().strftime("%B %d, %Y")
+ 'date': datetime.date.today().strftime("%B %d, %Y"),
+ 'r': model.decoder.r
}
best_loss = model_loss
bestmodel_path = 'best_model.pth.tar'
@@ -248,7 +250,7 @@ def set_init_dict(model_dict, checkpoint, c):
def setup_model(num_chars, num_speakers, c):
print(" > Using model: {}".format(c.model))
- MyModel = importlib.import_module('models.' + c.model.lower())
+ MyModel = importlib.import_module('TTS.models.' + c.model.lower())
MyModel = getattr(MyModel, c.model)
if c.model.lower() in ["tacotron", "tacotrongst"]:
model = MyModel(
@@ -305,3 +307,10 @@ def split_dataset(items):
else:
return items[:eval_split_size], items[eval_split_size:]
+
+def gradual_training_scheduler(global_step, config):
+ new_values = None
+ for values in config.gradual_training:
+ if global_step >= values[0]:
+ new_values = values
+ return new_values[1], new_values[2]
\ No newline at end of file
diff --git a/utils/radam.py b/utils/radam.py
new file mode 100644
index 00000000..62ecc695
--- /dev/null
+++ b/utils/radam.py
@@ -0,0 +1,154 @@
+import math
+import torch
+from torch.optim.optimizer import Optimizer
+
+
+# adapted from https://github.com/LiyuanLucasLiu/RAdam
+class RAdam(Optimizer):
+
+ def __init__(self, params, lr=1e-3, betas=(0.9, 0.999), eps=1e-8, weight_decay=0):
+ defaults = dict(lr=lr, betas=betas, eps=eps, weight_decay=weight_decay)
+ self.buffer = [[None, None, None] for ind in range(10)]
+ super(RAdam, self).__init__(params, defaults)
+
+ def __setstate__(self, state): # pylint: disable= useless-super-delegation
+ super(RAdam, self).__setstate__(state)
+
+ def step(self, closure=None):
+
+ loss = None
+ if closure is not None:
+ loss = closure()
+
+ for group in self.param_groups:
+
+ for p in group['params']:
+ if p.grad is None:
+ continue
+ grad = p.grad.data.float()
+ if grad.is_sparse:
+ raise RuntimeError(
+ 'RAdam does not support sparse gradients')
+
+ p_data_fp32 = p.data.float()
+
+ state = self.state[p]
+
+ if not state:
+ state['step'] = 0
+ state['exp_avg'] = torch.zeros_like(p_data_fp32)
+ state['exp_avg_sq'] = torch.zeros_like(p_data_fp32)
+ else:
+ state['exp_avg'] = state['exp_avg'].type_as(p_data_fp32)
+ state['exp_avg_sq'] = state['exp_avg_sq'].type_as(
+ p_data_fp32)
+
+ exp_avg, exp_avg_sq = state['exp_avg'], state['exp_avg_sq']
+ beta1, beta2 = group['betas']
+
+ exp_avg_sq.mul_(beta2).addcmul_(1 - beta2, grad, grad)
+ exp_avg.mul_(beta1).add_(1 - beta1, grad)
+
+ state['step'] += 1
+ buffered = self.buffer[int(state['step'] % 10)]
+ if state['step'] == buffered[0]:
+ N_sma, step_size = buffered[1], buffered[2]
+ else:
+ buffered[0] = state['step']
+ beta2_t = beta2 ** state['step']
+ N_sma_max = 2 / (1 - beta2) - 1
+ N_sma = N_sma_max - 2 * \
+ state['step'] * beta2_t / (1 - beta2_t)
+ buffered[1] = N_sma
+
+ # more conservative since it's an approximated value
+ if N_sma >= 5:
+ step_size = group['lr'] * math.sqrt((1 - beta2_t) * (N_sma - 4) / (N_sma_max - 4) * (
+ N_sma - 2) / N_sma * N_sma_max / (N_sma_max - 2)) / (1 - beta1 ** state['step'])
+ else:
+ step_size = group['lr'] / (1 - beta1 ** state['step'])
+ buffered[2] = step_size
+
+ if group['weight_decay'] != 0:
+ p_data_fp32.add_(-group['weight_decay']
+ * group['lr'], p_data_fp32)
+
+ # more conservative since it's an approximated value
+ if N_sma >= 5:
+ denom = exp_avg_sq.sqrt().add_(group['eps'])
+ p_data_fp32.addcdiv_(-step_size, exp_avg, denom)
+ else:
+ p_data_fp32.add_(-step_size, exp_avg)
+
+ p.data.copy_(p_data_fp32)
+
+ return loss
+
+
+class PlainRAdam(Optimizer):
+
+ def __init__(self, params, lr=1e-3, betas=(0.9, 0.999), eps=1e-8, weight_decay=0):
+ defaults = dict(lr=lr, betas=betas, eps=eps, weight_decay=weight_decay)
+
+ super(PlainRAdam, self).__init__(params, defaults)
+
+ def __setstate__(self, state): # pylint: disable= useless-super-delegation
+ super(PlainRAdam, self).__setstate__(state)
+
+ def step(self, closure=None):
+
+ loss = None
+ if closure is not None:
+ loss = closure()
+
+ for group in self.param_groups:
+
+ for p in group['params']:
+ if p.grad is None:
+ continue
+ grad = p.grad.data.float()
+ if grad.is_sparse:
+ raise RuntimeError(
+ 'RAdam does not support sparse gradients')
+
+ p_data_fp32 = p.data.float()
+
+ state = self.state[p]
+
+ if not state:
+ state['step'] = 0
+ state['exp_avg'] = torch.zeros_like(p_data_fp32)
+ state['exp_avg_sq'] = torch.zeros_like(p_data_fp32)
+ else:
+ state['exp_avg'] = state['exp_avg'].type_as(p_data_fp32)
+ state['exp_avg_sq'] = state['exp_avg_sq'].type_as(
+ p_data_fp32)
+
+ exp_avg, exp_avg_sq = state['exp_avg'], state['exp_avg_sq']
+ beta1, beta2 = group['betas']
+
+ exp_avg_sq.mul_(beta2).addcmul_(1 - beta2, grad, grad)
+ exp_avg.mul_(beta1).add_(1 - beta1, grad)
+
+ state['step'] += 1
+ beta2_t = beta2 ** state['step']
+ N_sma_max = 2 / (1 - beta2) - 1
+ N_sma = N_sma_max - 2 * state['step'] * beta2_t / (1 - beta2_t)
+
+ if group['weight_decay'] != 0:
+ p_data_fp32.add_(-group['weight_decay']
+ * group['lr'], p_data_fp32)
+
+ # more conservative since it's an approximated value
+ if N_sma >= 5:
+ step_size = group['lr'] * math.sqrt((1 - beta2_t) * (N_sma - 4) / (N_sma_max - 4) * (
+ N_sma - 2) / N_sma * N_sma_max / (N_sma_max - 2)) / (1 - beta1 ** state['step'])
+ denom = exp_avg_sq.sqrt().add_(group['eps'])
+ p_data_fp32.addcdiv_(-step_size, exp_avg, denom)
+ else:
+ step_size = group['lr'] / (1 - beta1 ** state['step'])
+ p_data_fp32.add_(-step_size, exp_avg)
+
+ p.data.copy_(p_data_fp32)
+
+ return loss
diff --git a/utils/speakers.py b/utils/speakers.py
index a1c273cf..4b11531b 100644
--- a/utils/speakers.py
+++ b/utils/speakers.py
@@ -1,7 +1,7 @@
import os
import json
-from datasets.preprocess import get_preprocessor_by_name
+from TTS.datasets.preprocess import get_preprocessor_by_name
def make_speakers_json_path(out_path):
diff --git a/utils/synthesis.py b/utils/synthesis.py
index 7d7bf604..f657eb4d 100644
--- a/utils/synthesis.py
+++ b/utils/synthesis.py
@@ -50,7 +50,7 @@ def parse_outputs(postnet_output, decoder_output, alignments):
return postnet_output, decoder_output, alignment
-def trim_silence(wav):
+def trim_silence(wav, ap):
return wav[:ap.find_endpoint(wav)]
@@ -114,5 +114,5 @@ def synthesis(model,
wav = inv_spectrogram(postnet_output, ap, CONFIG)
# trim silence
if do_trim_silence:
- wav = trim_silence(wav)
+ wav = trim_silence(wav, ap)
return wav, alignment, decoder_output, postnet_output, stop_tokens
diff --git a/utils/text/__init__.py b/utils/text/__init__.py
index 332163d2..1c5b98c3 100644
--- a/utils/text/__init__.py
+++ b/utils/text/__init__.py
@@ -3,8 +3,8 @@
import re
import phonemizer
from phonemizer.phonemize import phonemize
-from utils.text import cleaners
-from utils.text.symbols import symbols, phonemes, _phoneme_punctuations, _bos, \
+from TTS.utils.text import cleaners
+from TTS.utils.text.symbols import symbols, phonemes, _phoneme_punctuations, _bos, \
_eos
# Mappings from symbol to numeric ID and vice versa:
@@ -17,7 +17,7 @@ _ID_TO_PHONEMES = {i: s for i, s in enumerate(phonemes)}
# Regular expression matching text enclosed in curly braces:
_CURLY_RE = re.compile(r'(.*?)\{(.+?)\}(.*)')
-# Regular expression matchinf punctuations, ignoring empty space
+# Regular expression matching punctuations, ignoring empty space
PHONEME_PUNCTUATION_PATTERN = r'['+_phoneme_punctuations+']+'
@@ -47,7 +47,7 @@ def text2phone(text, language):
def pad_with_eos_bos(phoneme_sequence):
- return [_PHONEMES_TO_ID[_bos]] + phoneme_sequence + [_PHONEMES_TO_ID[_eos]]
+ return [_PHONEMES_TO_ID[_bos]] + list(phoneme_sequence) + [_PHONEMES_TO_ID[_eos]]
def phoneme_to_sequence(text, cleaner_names, language, enable_eos_bos=False):
diff --git a/utils/text/symbols.py b/utils/text/symbols.py
index 9b7a36b4..ee6fd2cf 100644
--- a/utils/text/symbols.py
+++ b/utils/text/symbols.py
@@ -18,7 +18,7 @@ _vowels = 'iyɨʉɯuɪʏʊeøɘəɵɤoɛœɜɞʌɔæɐaɶɑɒᵻ'
_non_pulmonic_consonants = 'ʘɓǀɗǃʄǂɠǁʛ'
_pulmonic_consonants = 'pbtdʈɖcɟkɡqɢʔɴŋɲɳnɱmʙrʀⱱɾɽɸβfvθðszʃʒʂʐçʝxɣχʁħʕhɦɬɮʋɹɻjɰlɭʎʟ'
_suprasegmentals = 'ˈˌːˑ'
-_other_symbols = 'ʍwɥʜʢʡɕʑɺɧ '
+_other_symbols = 'ʍwɥʜʢʡɕʑɺɧ'
_diacrilics = 'ɚ˞ɫ'
_phonemes = sorted(list(_vowels + _non_pulmonic_consonants + _pulmonic_consonants + _suprasegmentals + _other_symbols + _diacrilics))
diff --git a/utils/visual.py b/utils/visual.py
index 982fa53a..ab513666 100644
--- a/utils/visual.py
+++ b/utils/visual.py
@@ -1,14 +1,19 @@
+import torch
import librosa
import matplotlib
matplotlib.use('Agg')
import matplotlib.pyplot as plt
-from utils.text import phoneme_to_sequence, sequence_to_phoneme
+from TTS.utils.text import phoneme_to_sequence, sequence_to_phoneme
-def plot_alignment(alignment, info=None):
- fig, ax = plt.subplots(figsize=(16, 10))
+def plot_alignment(alignment, info=None, fig_size=(16, 10), title=None):
+ if isinstance(alignment, torch.Tensor):
+ alignment_ = alignment.detach().cpu().numpy().squeeze()
+ else:
+ alignment_ = alignment
+ fig, ax = plt.subplots(figsize=fig_size)
im = ax.imshow(
- alignment.T, aspect='auto', origin='lower', interpolation='none')
+ alignment_.T, aspect='auto', origin='lower', interpolation='none')
fig.colorbar(im, ax=ax)
xlabel = 'Decoder timestep'
if info is not None:
@@ -17,12 +22,18 @@ def plot_alignment(alignment, info=None):
plt.ylabel('Encoder timestep')
# plt.yticks(range(len(text)), list(text))
plt.tight_layout()
+ if title is not None:
+ plt.title(title)
return fig
-def plot_spectrogram(linear_output, audio):
- spectrogram = audio._denormalize(linear_output)
- fig = plt.figure(figsize=(16, 10))
+def plot_spectrogram(linear_output, audio, fig_size=(16, 10)):
+ if isinstance(linear_output, torch.Tensor):
+ linear_output_ = linear_output.detach().cpu().numpy().squeeze()
+ else:
+ linear_output_ = linear_output
+ spectrogram = audio._denormalize(linear_output_) # pylint: disable=protected-access
+ fig = plt.figure(figsize=fig_size)
plt.imshow(spectrogram.T, aspect="auto", origin="lower")
plt.colorbar()
plt.tight_layout()